Reinforcement Learning for A/B Testing Platform
Launching hundreds of A/B testing campaigns, learns and iterates over the resulting statistics to reach an arbitrary set of campaign goals.
16 Aug 2019
4 min read
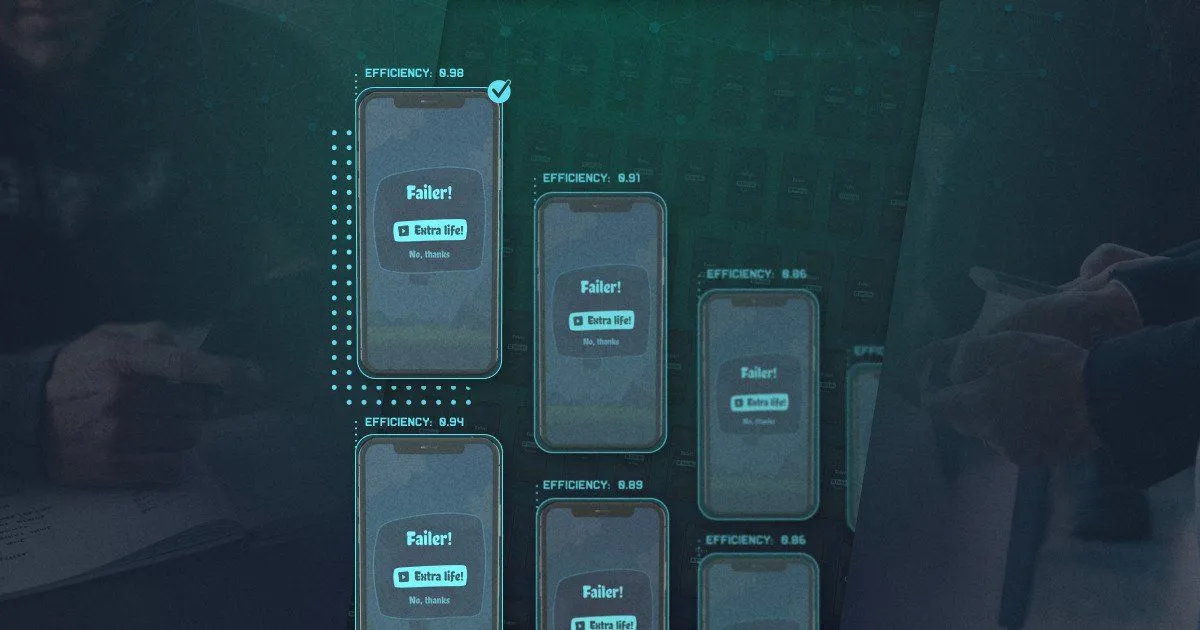
Summary
- Deciding on which game updates take priority should be a data-driven decision. The impact of any change on the user activity and game revenue are often measured through A/B testing.
- A large gaming company requested a self-learning platform which automatically creates and launches hundreds of A/B testing campaigns, learns and iterates over the resulting statistics to reach an arbitrary set of campaign goals.
Tech Stack
Firebase
OpenAI Gym
Python
TensorFlow
Timeline
1 Week
Solution Architecture Design
Solution Architect
2 Weeks
Hypothesis Generation & Validation
Deep Learning Researcher
4 Weeks
RL Environment Development
Data Engineer
Deep Learning Engineer
Deep Learning Researcher
2 Weeks
RL Algorithms Development
Deep Learning Researcher
6 Weeks
Training & Tuning Cycle pt.1
Deep Learning Researcher
2 Weeks
Data Labelling & Processing & Integration into RL Environment
Data Engineer
2 Weeks
Copy of Training & Tuning Cycle pt.2
Deep Learning Researcher
2 Weeks
Integration & A/B Testing & Deployment
Backend Developer
Dev Ops
Tech Challenge
- When tested product goes through various stages of its life-cycle, goals might swap priorities.
- Most of the tests are created manually and managed by humans, with only few parameters changed at once not to introduce over-complexity into results’ interpretation.
- Different parameters, competing goals, lots of statistical events are the factors that introduce additional complexity and need automatic insights extraction.
- Finding complex interaction between the entire list of A/B parameters and real-world state is extremely technically challenging.
Solution
- Our team used recently introduced techniques from reinforcement and deep learning to model complex parameters interaction, time dependent external state and A/B campaign events stream.
- As a result, we have developed a self-learning solution which, firstly, pre-trains itself using historical data and then takes control over the campaigns. Product owner remains in control of goals and parameters.
- System creates hundreds of micro-campaigns with different goals and fast-checks hypotheses on a user base sample. Then, it explores the resulting space with deeper tests to maximize the outcomes in each cycle.
- Campaign results are integrated back via learning process and checked against updated goals; the cycle repeats.
- AI explores sophisticated dependencies in parameters/state spaces and their relations with the list of goals, provides opportunities beyond conventional approach to A/B testing, while the rest is controlled by product owner in an intuitive way.
Impact
- First, we tested, validated and then applied the approach to the mobile game application.
- Then we have compared our solution to Bayesian optimization reference and found that our reinforcement learning solution shows superior results to Bayesian baseline.
- Furthermore, the self-learning solution is adaptable to completely different cases with relative ease.
- Full results presentation is available upon request.
Have an idea? Let's discuss!
Book a meeting
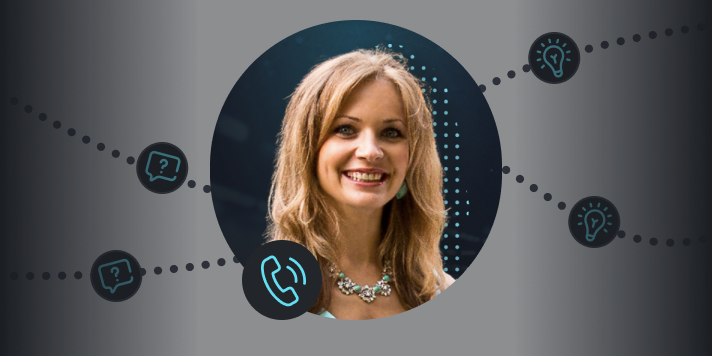
Yuliya Sychikova
COO @ DataRoot Labs
Do you have questions related to your AI-Powered project?
Talk to Yuliya. She will make sure that all is covered. Don't waste time on googling - get all answers from relevant expert in under one hour.
Talk to Yuliya. She will make sure that all is covered. Don't waste time on googling - get all answers from relevant expert in under one hour.
OR
Important copyright notice © DataRoot Labs and datarootlabs.com, 2024. Unauthorized use and/or duplication of this material without express and written permission from this site’s author and/or owner is strictly prohibited. Excerpts and links may be used, provided that full and clear credit is given to DataRoot Labs and datarootlabs.com with appropriate and specific direction to the original content.