Dynamic Pricing Algorithms on Uber and Lyft
Establishing a robust and reliable dynamic pricing system.
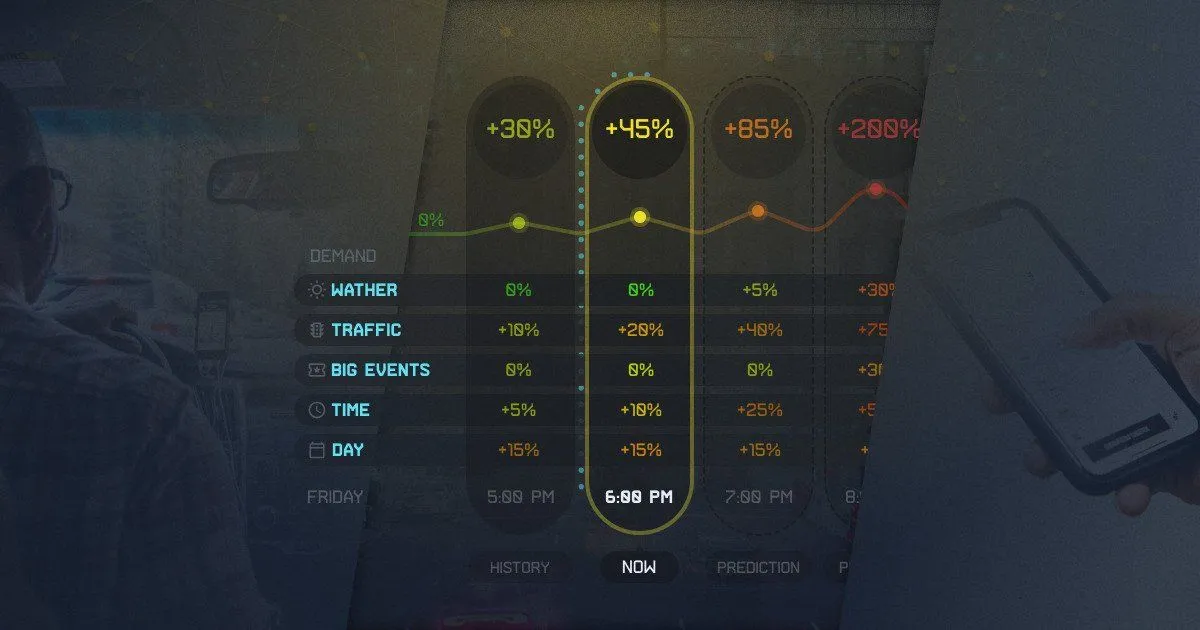
Without doubts, dynamic pricing algorithms lie at the core of Uber and Lyft market success, especially since they solve a multitude of problems while simultaneously benefiting both the industries and the customers (at least, on paper). To fully understand the advantages of such an e-commerce practice, however, it may be helpful to figure out how the dynamic pricing works, to see its advantages and disadvantages, as well as to delve into the concrete business cases and the other industries where the technique makes an overreaching impact.
The Essence of Dynamic Pricing Algorithms
Directly speaking, dynamic pricing functions accordingly to some basic economic principles. If the relative supply becomes scarce and the demand is high, then there is an apparent need for raising the overall price to match the supply/demand curves. Because all of the businesses need constant revenue, dynamic pricing makes a perfect sense, especially in the long-term.
Dynamic pricing merely ensures that there is a constant supply of the demanded things (whether it is a physical product or a call for service) due to the incentive-based system. All the while, dynamic pricing also guarantees that all the involved parties receive the option, or a chance so to speak, of obtaining what they want, regardless of the possible circumstances.
Advantages and Disadvantages of Dynamic Pricing
As for the relative pros and cons, it’s vital to comprehend the notion that the seller usually wins: dynamic pricing algorithms ideally allow for maximizing the profits out of every single client. The seller can establish some discriminatory policies that are going to reflect his or her subjective overview of the market, even though the customers may realize that they are overpaying for the same solution, factually speaking. Such an approach may turn away some of the clients, yet still, allow the seller to receive the utmost benefit. Otherwise, if the demand for the product is relatively low, it’s still possible to generate some revenue by offering promotions for appreciating the general supply/demand chart. In such a scenario, it will be the customer who “gains” the most. Even though the real-life models may contain slightly more variables that influence the pricing, it always comes down to these rooting rules and principles.
Uber Dynamic Pricing Algorithm: a Pure Example
In its entity, Uber relies extensively on machine learning (ML) to establish a robust and reliable dynamic pricing system. With the help of ML, Uber generates a future-aware forecast of multiple conditions of the market and uses a system that is very sensitive to external factors: these factors ultimately include the global news events, weather, historical data, holidays, time, traffic, etc.
However, what happens if there is not enough information for making a prediction? When there is little or no data at all, Uber exploits LSTM (long short-term memory) networks as well as deep learning models to predict the future stance of the market and even the “unexpected” events before they can happen.
To clarify, LSTM’s are building blocks for layers of a recurrent neural network (RNN), the one that allows information to persist. All of the LSTM’s are adapted for classifying, learning, and predicting time series, even if there are time lags between important events. By using LSTM’s, Uber ultimately allows itself to effectively calculate and analyze all of the needed variables to form a price that would reflect the current state of the market and optimize the overall experience.
lyft Dynamic Pricing Algorithm: Another One in the Bucket
Lyft, on the other hand, seems to combat the market share of Uber with its vision of dynamic pricing: instead of indefinitely appreciating the prices to meet the real market needs, Lyft positioned that fares should be somewhat fixed and relatively reflect the existing demand. To this end, the Lyft pricing algorithms uses machine learning to analyze multiple variables, such as traffic and weather, for instance, to ultimately determine the demand for the drivers. As a result, Lyft created two distinct features that shape the overall price. One of them, called the Prime Time, increases the fare at two hundred percent when there is a broad request for the rides out there. On the opposite, the Happy Hour, ultimately decreases the overall price up to 50% when there is a low demand for the trips.
Dynamic Pricing Algorithm at Other Industries
The fuel industry is an ideal illustration of dynamic pricing and all of its implications. The price of petroleum-based fuels differs from place to place and is dependent on the popularity of a particular gas station, the oil prices, and the consumer buying power in some of the cases. In turn, it’s incredibly difficult to know in advance the cost of fuel on the day-to-day basis as it undergoes the permanent changes to ultimately meet the demand and adequately reflect the market’s needs.
The other pure example of dynamic pricing would be the airline industry where the prices can change in a matter of seconds. Sometimes, it’s not uncommon for the one-way tickets to exceed the cost of the round-trip or for the 2000-mile trip to be less expensive than its 200-mile counterpart. There are lots of variables to be analyzed to determine the reasons behind such a price formation, yet it’s clear that the airline industry is no exception in using dynamic pricing for benefitting the most in every single scenario.
Whether it is an entertainment, a hotel, a retail, a travel, or manufacturing industries, one can be confident that dynamic pricing algorithms are always in action due to its practicality and profitability.