The Next Era of AI-Powered Marketing Conversations
A discussion with Neha Mittal on how Just Words is enabling bidirectional marketing and shaping the future of personalized content delivery.
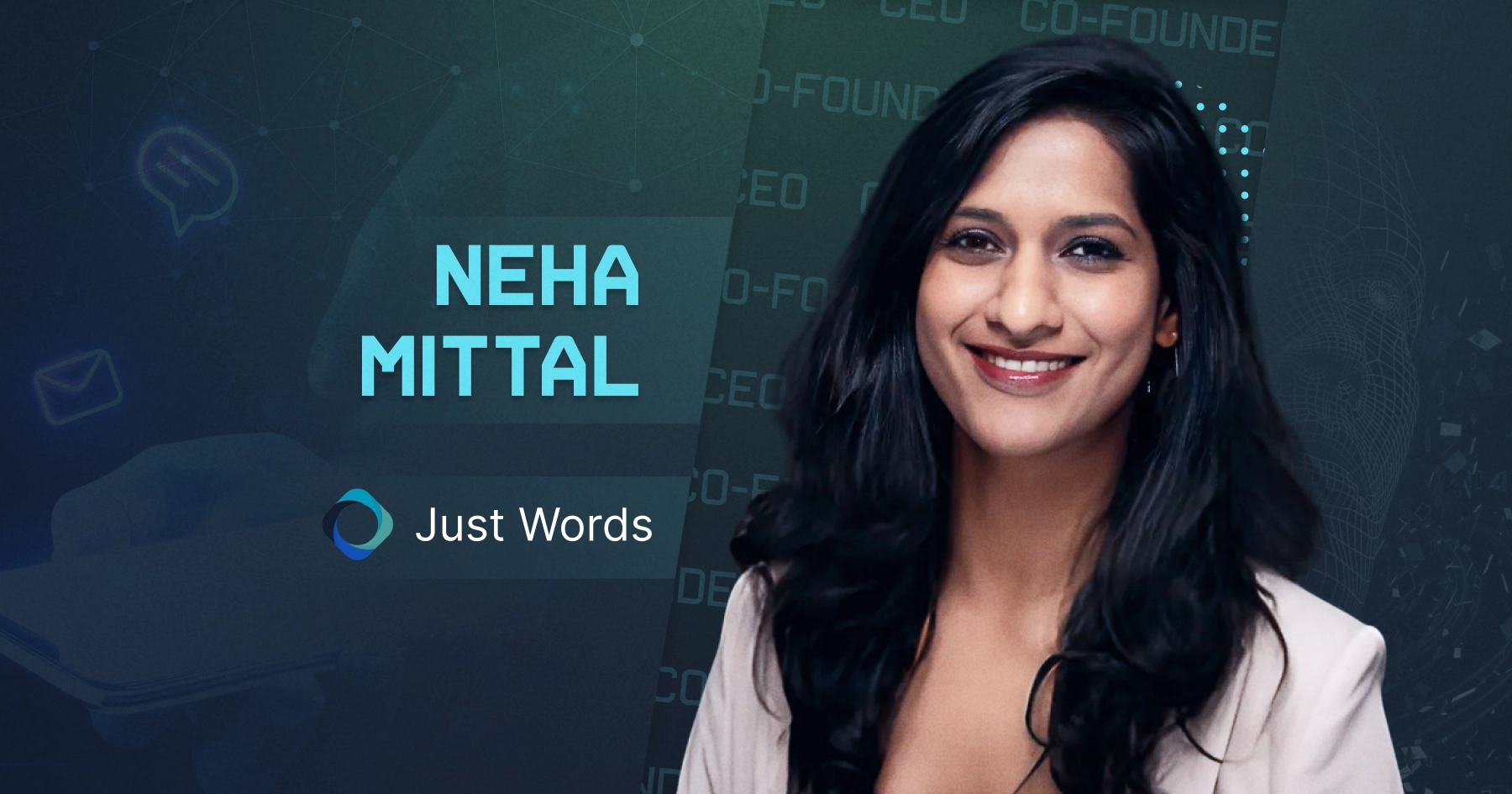
Neha Mittal is the CEO and Co-Founder of Just Words, a US company that helps companies boost user retention by dynamically refreshing content on emails, push, in-app messages, and websites. It is built on a modern stack that replaces old-school campaign marketing with an ML-first approach to content personalization. Just Words develops an AI-powered platform designed to optimize product copy for businesses to boost user growth. Their core product auto-refreshes content on growth channels like emails by leveraging reinforcement learning, AI, and causal inferences.
Founded in the US in 2024, Just Words raised $2.2M in a Seed Round led by Cloud Capital and Peak XV Partners.
Just Words aims to transform content into conversations using AI. Can you elaborate on how your platform utilizes reinforcement learning to achieve hyper-personalized messaging?
Stepping back a little bit. Today, we think of hyper-personalized messaging as something more ad hoc, like, “Let's use AI to create random content and see if it works.”
We use reinforcement learning to build a continuous loop of creating better content over time. Here's how RL works: we send content to you and track your actions — did you open the email? Did you click on it? Did you buy something after receiving the message? We analyze that data to understand what and how much to send you next time.
Reinforcement learning comes into play when we start adjusting content delivery based on user reactions. Over time, we may observe that males engage more with certain content than females. If we notice this pattern, we'll direct more traffic to males for that content. This is how we automate hyper-personalized messaging, eliminating the need to manually guess how to link and target users.
We use reinforcement learning to build a continuous loop of creating better content over time. This is how we automate hyper-personalized messaging, eliminating the need to manually guess how to link and target users.
Just Words has helped companies accelerate their speed of experimentation tenfold. What challenges did you face in developing this capability, and how did reinforcement learning contribute to overcoming them?
There are many factors, even in resource planning, that need to be done correctly.
Here's how it works: you start with one piece of content and create hundreds of variations, then present those variations to users simultaneously. Instead of testing one at a time, we use dynamic or pattern testing, allowing us to test 10 pieces of content at once. This approach gives you quick results on what's working and what's not. I'd much rather do that than wait three weeks for every test.
The next challenge is supporting the volume. Since users are now divided into, say, 10 variations instead of just one, we need to ensure enough traffic for meaningful results. If the volume is too low, we risk getting static or unreliable results.
To solve this, instead of sending traffic to all variations, we built a system that selects a few variations based on the volume of users. The system is smart enough to test just two or four at a time, and with reinforcement learning, it quickly identifies the winners and losers.
When something works, reinforcement learning drives more traffic there. If something's underperforming, traffic is reduced. This helps us do two things: first, we quickly identify the best-performing variants, and second, we reduce dilution effects. For example, when testing 10 variants, if 9 are bad and 1 is good, our system ensures the good variant stands out.
If all users are exposed to the 9 bad variants, that's detrimental. But with reinforcement learning, we minimize traffic to those bad variants and make sure most users see the one good variant. This method allows us to test efficiently while still ensuring positive, reliable metrics over time.
In your journey from founding CityStructure to leading Just Words, what key lessons have you learned about integrating AI into marketing strategies?
Both at Twitter and Pinterest, I was focused on growth and managing things like push notifications. There were a few key learnings. One was around the operational process of sending notifications. Typically, you'd send one email, wait two days, then follow up with another email and a push notification two days later. It's a manual process—you have to build and monitor each step, which could require a 20-person team to manage effectively. At Twitter, we eliminated all of that. We built an engine that predicts the best message and timing for delivery.
Who should receive which message? We built a machine learning infrastructure to answer that. To me, AI is about feeding the system the right data to deliver the most relevant content based on what you know about your users.
At Twitter and Pinterest, machine learning was essential — so I knew it could be a valuable product for other companies. But building it takes time and a few engineers, and not every company has the resources or capacity to do that.
By building it this way, we gain operational efficiency and better data for personalization. Humans make complex judgments with limited data, but machines do it at scale. Before AI, handling language complexity was tough—now, AI can scale while understanding what resonates with users.
Here's a quick example from Twitter: our biggest bottleneck in showing the best message was copy creation. We had only one or two copywriters for the entire growth team, so we were limited to testing whatever they could produce — and writing compelling copy is hard.
This is where we were slow. Now, we're saying we can just feed some understanding to the AI, and it will understand what content not to generate. So it's such a big unlock, not only to create content but also for speeding up testing.
Can you share a specific example where Just Words' AI-driven approach led to a substantial increase in user engagement for a client?
Grammarly is an interesting one. When we first started with them, they were testing maybe one or two changes in emails to see whether they were working.
Our first few tests with them focused on questions like: Can we upsell free users to premium? Would offering a discount improve conversions? Previously, they weren't adjusting the discount amount—they sent the same email to all users, regardless of their differences. We made simple changes, like tailoring variations for students versus professionals.
Students typically want a discount, while professionals are looking for a different kind of value. So, we tailored the tone and messaging for each group. Over two weeks, we tested 11 to 15 variations. Using reinforcement learning, we then optimized by showing students what resonated most with them and did the same for professionals. Over time, we identified the top-performing variations for each group, which led to a 7.3% increase in free-to-paid subscriptions. That was a big win for Grammarly. In essence, we tested personas and learned what worked best for each to drive results.
Now, a very different example — BeReal, a social media company operating across Europe. Their challenge was language diversity. They were only testing content in English, then directly translating it into other languages without validating performance in each market. This led to two problems: poor-quality translations and no insight into whether the messaging actually resonated.
We ran 60 variants across Spanish, French, German, and Italian and found that each language had a different top performer. What worked in English didn't translate to success elsewhere. We also uncovered fatigue effects — messaging that performed well initially lost effectiveness as new options were introduced.
For a company like BeReal, where users crave novelty in their notifications, this was a key insight. It's a different use case, but through testing and reinforcement learning, we were able to uncover valuable patterns and improve results.
The human-in-the-loop approach ensures that only high-quality content reaches users.
How does Just Words ensure that its AI models remain ethical and avoid issues like strategic deception, which have been observed in some reinforcement learning systems?
Our tagline emphasizes that AI gives users control. The idea isn't to let AI run wild—we're intentional about avoiding content that's inaccurate, off-brand, disrespectful, or misleading.
Our product uses AI to generate content variations at scale—across segments, formats, and more. But nothing goes live without human review. You get to approve or reject each variation, with feedback like: “This didn't work, here's why,” or “This looks good, I'll approve it.”
This human-in-the-loop approach ensures that only high-quality content reaches users. That feedback also trains the model, teaching it how to speak in your brand's voice and avoid errors or misleading language—like what we did with Grammarly. It's all about making sure the content is accurate and respectful and resonates with users.
What is your ideal customer profile?
Right now, our ideal customers are larger B2C or B2B companies with a solid foundation—companies that have a CRM in place and are already sending emails but haven't yet tackled personalization. Global companies with localization needs also fit well. These are our sweet spots today.
In the future, we'll expand to mid-market and smaller companies. As they build their foundations, they can start with a personalization-first approach without needing to retrofit existing systems. SMBs won't have to go back and say, “Oh, I built it this way, now how do I correct it?” They will be personalized. But for now, our focus is on larger companies that are ready to take the next step.
Looking ahead, what are your aspirations for Just Words in terms of leveraging AI to further revolutionize content marketing?
Today, marketing teams are often large because even simple personalization, like distinguishing between students and professionals, requires manually creating branches, scheduling emails, and waiting days between follow-ups. It's a time-consuming, manual process.
And that’s just the start. After emails are sent, teams wait for results, which then need to be analyzed by data scientists. Based on those results, the next test is set up, often on the fly. Marketers are left guessing what resonates, what to try next, and how to scale it. It's inefficient and frustrating and drains resources. At scale, it becomes chaotic. There's no clear method — just “whatever seems to make sense.”
We envision a different world. As a marketer, you have the deepest knowledge about your product, users, goals, and growth plans. You feed that into the system, and from there, the system becomes your marketer. It handles the heavy lifting — crafting messages, structuring email flows, deciding timing, and choosing between email or push — all automatically.
And it doesn't just work silently in the background. Every week or two, it proactively updates you: “Here's what I learned. Here's what worked. Want to apply this to your next strategy?” It becomes like a helpful teammate, constantly learning, testing, and evolving while keeping you in the loop so you can focus on strategy, not operations.
That's one part of how we see AI transforming marketing. The second part is shifting marketing from one-directional to truly bidirectional. Today, marketing is mostly broadcast — companies send emails, and that’s the end of it. But we're moving toward a world where every interaction is a two-way conversation.
Instead of just tracking opens or clicks, we'll respond to what people actually say. Messages will adapt in real time based on user feedback. It becomes hyper-personalized communication where marketing and sales begin to merge, and every message leads to a smarter, more relevant follow-up.
That's the future we're building.