Personalization with AI: A Conversation with Kevin Kahn, CEO of Albatross
Explore how Albatross redefines personalization by adapting to user interests and catalog attributes.
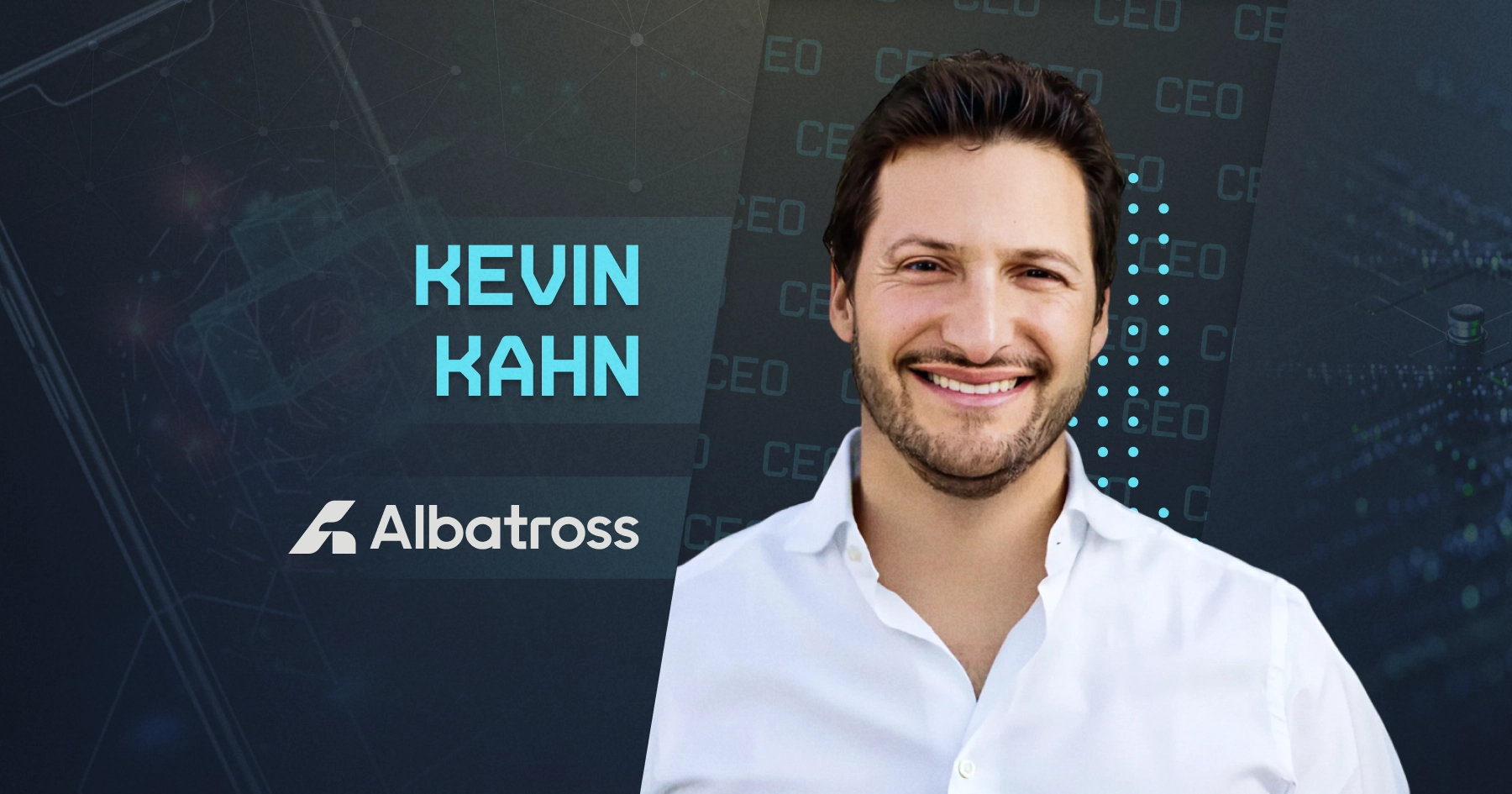
Kevin Kahn is the CEO and Co-Founder of Albatross, a Swiss company that empowers businesses to deliver real-time, personalized user experiences with the most advanced AI ranking engine. Albatross uses deep learning and reinforcement learning to generate and rank content in real-time, maximizing in-session engagement. Unlike traditional recommendation systems that rely on popularity and user similarity — often leading to churn — Albatross adapts to evolving user interests and dynamic catalog attributes like price. Founded in Switzerland in 2024, Albatross raised EUR 3M in a Pre-Seed round within 4 weeks of incorporation, led by Redalpine with participation from Daphni.
Albatross uses AI to create a personalized user experience. Can you speak to what specific pain points traditional recommendation systems face that Albatross solves?
Traditional systems relying on popularity-based rankings and user similarity fail to adapt to evolving interests and rapidly changing catalogs, leading to churn and lost revenue. These methods often create "rich-get-richer" dynamics, where popular items get recommended repeatedly while much of the catalog remains undiscovered. Dynamic factors like pricing and product availability further exacerbate these issues, leaving much of the catalog undiscovered and resulting in high churn rates.
Albatross solves these pain points by doing one thing extremely well: we developed the most advanced AI ranking engine. We leverage in-session user behavior and dynamic catalog attributes to deliver fresh, relevant, and highly personalized recommendations that evolve with each user’s preferences in every session. Powered by deep learning and sophisticated reinforcement learning, our technology is built on two key pillars:
- Real-Time Learning: Instantly adapts to user interactions, ensuring content is relevant without necessarily relying on prior user data.
- Intelligent Ranking: Dynamically positions products, images, and promotions to maximize visibility, engagement, and conversions.
Having led some of the largest machine learning and recommendation platforms at Amazon, what were some of the key learnings you've carried to Albatross, and how do those inform your approach to AI-driven personalization?
At Amazon, we discovered three important things:
- Ranking is the cornerstone of effective personalization, not candidate selection, which traditional recommendation engines do. Engagement uplift primarily comes from orchestrating content in a contextually relevant manner rather than simply selecting a subset of items, even if some are the right ones (as users will not see them).
- The critical role of in-session signals is to capture real-time intent. Even the most advanced systems today only rely on historical behavior, which is limiting, especially for anonymous users or those who rarely return. An example of where in-session data is successfully used is YouTube. For instance, if you usually watch the news but start exploring movie trailers, the algorithm quickly pivots to show more trailers, reflecting your in-session interests. The next day, these suggestions fade, and new content emerges based on fresh interactions.
- The importance of simplicity: a single, general-purpose ranker that captures customer signals across all touchpoints consistently outperforms bespoke personalization workflows tailored to individual experiences. Traditional models often fall short when dealing with rapidly changing user behavior and dynamic catalogs.
At Albatross, we’ve developed a state-of-the-art ranking engine that doesn’t just rely on historical data but also dynamically adapts to in-session interactions. Our contextual multi-armed bandit algorithms continuously learn and balance exploration with exploitation, ensuring recommendations are always fresh and relevant.
Albatross uses some of the most advanced deep learning and reinforcement learning techniques. How do these technologies work to enhance real-time user interactions?
The Albatross AI Ranking Service (ARES) dynamically optimizes item rankings based on real-time user interactions (events), historical session data, and dynamic catalog attributes. ARES comprises four key components that work together to produce context-aware, optimized recommendations:
- User Layer: Captures predictive user affinities based on sequences of user interactions.
- Catalog Layer: Factors in dynamic product details such as pricing, availability, and content freshness.
- Ranking Layer: Computes the optimal order of items.
- Feedback Loops: Continuously fine-tunes recommendations based on real-time engagement data.
Our system uses advanced learning-to-rank algorithms and contextual multi-armed bandits (MABs) to provide:
- a scoring logic to predict the likelihood of user conversion with a recommended item,
- an exploration logic to enhance future predictions with new data, and
- a debasing logic to assess true item relevance, regardless of their position.
This architecture ensures that recommendations evolve with the user, maximizing engagement and conversion rates.
In October 2024, Albatross raised a EUR 3M funding round led by Redalpine, with participation from Daphni. How will you use this investment?
The funding enables us to attract top talent and accelerate product development. Albatross isn’t just about building the best models; it’s about creating a platform that makes deploying these models effortless. We’re investing in simplifying integrations to reduce deployment times, minimizing technical overhead, and ensuring ultra-low latency at scale. This allows businesses to realize ROI faster, without the complexity typically associated with AI-driven personalization.
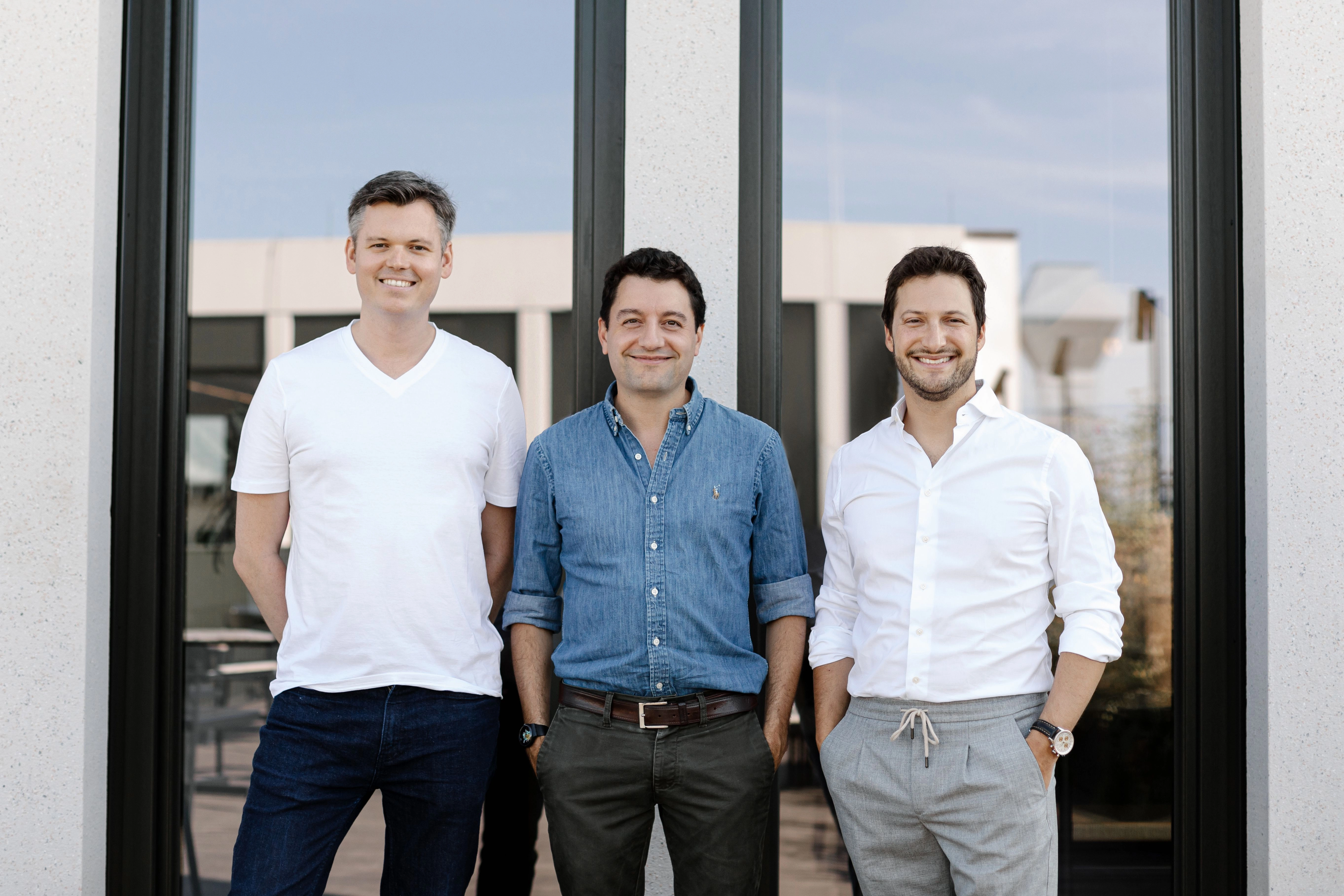
Albatross founders (left to right): Johan Boissard, Matteo Ruffini, Kevin Kahn
Your platform focuses on providing personalized experiences without invasive tracking methods. How does Albatross balance effective personalization with user privacy concerns?
Our real-time learning models adapt to user behavior within sessions, reducing reliance on invasive long-term data storage. We prioritize privacy by processing aggregated, pseudonymized data, ensuring compliance with GDPR and other global data protection regulations. This approach enables us to deliver personalized experiences without compromising user privacy.
Could you share some examples of how Albatross's AI-driven solutions have transformed user engagement for businesses in sectors like e-commerce and online media?
We are currently in the integration phase with several customers and have already delivered 10+ million predictions in January alone. Early results show double-digit improvements in click-through rates against handcrafted baselines. As we complete onboarding with these customers, we’ll share specific success stories.
What is the most challenging aspect of building and deploying AI-powered personalization solutions, and how do you think Albatross has managed to break these barriers?
The biggest challenge is reducing implementation time to deliver measurable ROI quickly. Many SaaS solutions require months of integration and even longer to show meaningful impact. At Albatross, we've reduced this time significantly. With current clients, we've demonstrated measurable results within 3–6 months, depending on the project scope. Our goal is to shorten this even further by evolving into a self-serve platform, enabling businesses to deploy personalization effortlessly without extensive technical support.
What are your strategic goals for the next few years, and what potential impact might this have on the AI personalization landscape?
Our ambition is to establish Albatross as the go-to personalization engine for any company looking to orchestrate content effectively. In an era where foundational models are the gateway to content, our relevance grows exponentially: we don’t just help users access content; we ensure it’s organized, ranked, and presented in the most engaging way for each individual. In a nutshell, we want to be the invisible engine behind how the world’s content is discovered, experienced, and monetized.
Our growth strategy focuses on three key areas:
- Market Development: Expanding our customer base within target verticals.
- Customer Growth: Empowering existing customers to deploy Albatross across more use cases.
- Product & Market Expansion: Entering new verticals and launching additional products.