Cancer Detection with AI
Emerged AI Models for Effective Diagnosis and Treatment.
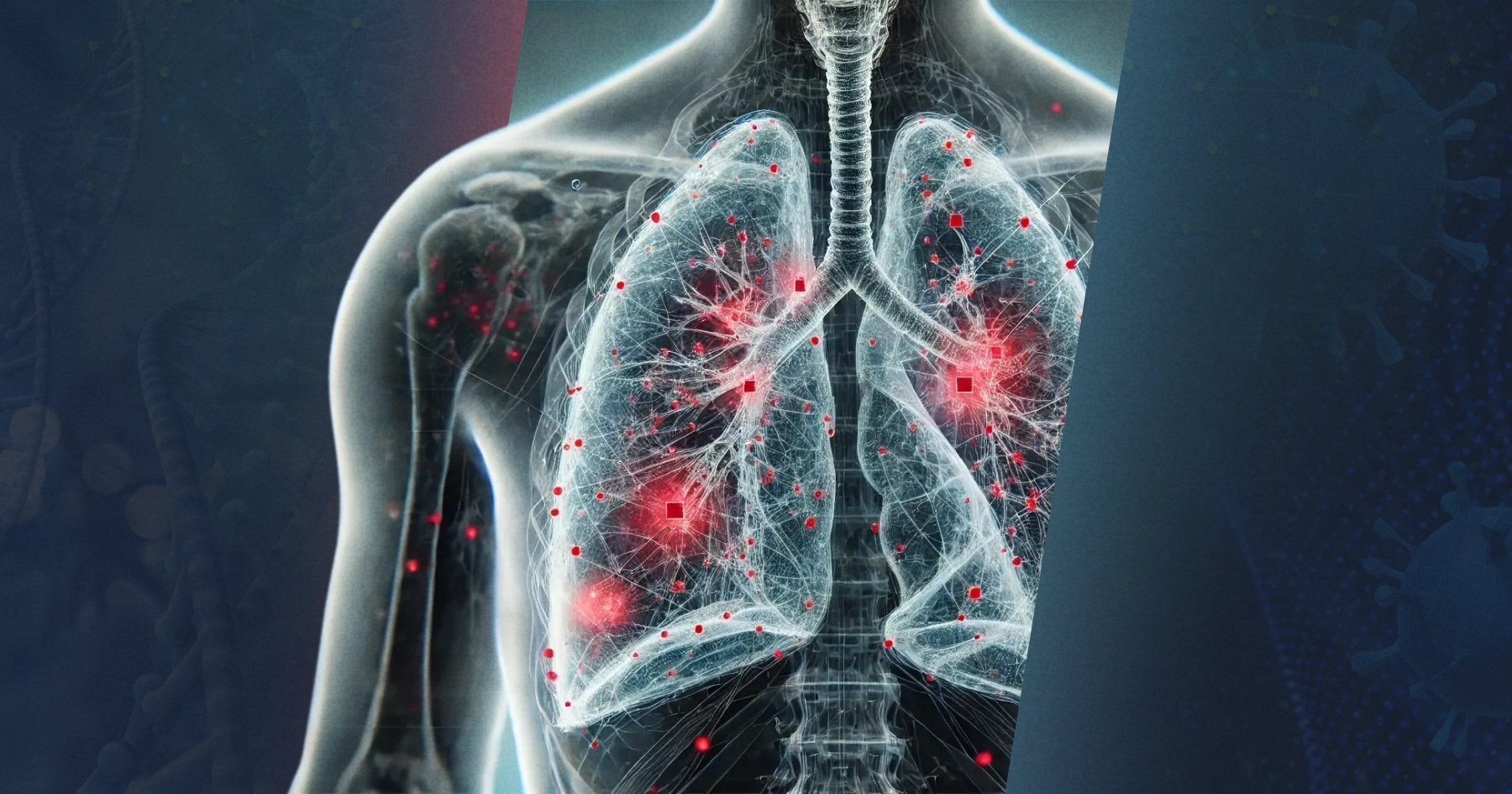
Cancer…the word that makes almost every person shiver. It remains one of the leading causes of death worldwide, with early detection being a crucial point for improving patient outcomes. In the rapidly evolving era of technologies, while scientists are working persistently to find a cure for cancer, artificial intelligence makes its pivotal contribution to diagnostics and treatment.
In this article, we will explore how AI transforms the landscape of cancer diagnosis, offering new tools that enhance accuracy, speed, and accessibility. At the moment, we are not talking about replacing physicians or scans. Instead AI tools emerge to play the role of a smart co-pilot, assisting physicians call the shots. Let's look at the models that are already in play showing promising results.
The 2 new foundation models, UNI and CONCH, developed by Harvard Medical School researchers at Brigham and Women's Hospital are advanced AI systems designed for medical applications, particularly in pathology. Their code has been made publicly available for further research.
UNI specializes in interpreting pathology images and can recognize diseases in specific regions on slides and whole-slide images. Trained on over 100M tissue patches and over 100K whole-slide images, UNI excels at disease detection, diagnosis, and prognosis, especially in cancer classification and organ transplant assessment.
CONCH is a multimodal model that can analyze both pathology images and corresponding text. Trained on more than 1.17M image-text pairs, CONCH can identify rare diseases and distinguish cancerous and benign tissues, assessing tumor growth and spread. It allows pathologists to interact with the model via text queries, making it useful for searching images for specific features.
The CHIEF (Clinical Histopathology Imaging Evaluation Foundation) foundational model was trained on 15M unlabeled images and further refined on 60K whole-slide images from various tissues, enabling it to consider both specific regions and overall context for comprehensive interpretation. In 5 biopsy datasets, CHIEF achieved 96% accuracy across cancers like esophagus, stomach, colon, and prostate. When tested on unseen slides from tumors in the colon, lung, breast, endometrium, and cervix, it maintained over 90% accuracy.
Validated on 19K+ images from 32 datasets across 24 hospitals, CHIEF outperformed other AI methods by up to 36% in cancer cell detection, tumor origin identification, outcome prediction, and identifying genes linked to treatment response. The model also distinguished patients with longer-term survival from those with shorter-term survival, outperforming other models by 8%. Future plans include expanding CHIEF's capabilities to rare diseases, pre-malignant tissues, and predicting responses to novel cancer treatments.
Researchers from the University of Gothenburg developed an AI model named Candycrunch that improves cancer detection by analyzing sugar structures (glycans). Trained on 500K+ glycan structures, Candycrunch determines the exact sugar structure in 90% of cases within seconds. This quick analysis speeds up the identification of glycan-based biomarkers, crucial for early cancer diagnosis, and allows for the discovery of previously undetectable biomarkers, opening new opportunities for cancer biology research and personalized treatments.
Paige, in collaboration with Microsoft, introduced Virchow2 and Virchow2G, the second generation of its foundation model for cancer, surpassing all other pathology models in scale. Built on a dataset of over 3M pathology slides from 800+ labs in 45 countries, these models include data from over 225K patients, covering over 40 different tissue types. With 1.8B parameters, Virchow2G is the largest pathology model ever created. Their inclusion of various genders, races, ethnicities, and geographical regions contributes to a comprehensive understanding of cancer.
These models were trained using Microsoft's supercomputing infrastructure, tripling the size of previous models. They aid in cancer detection across various tissue types, improving the accuracy of diagnoses. Additionally, Paige's AI modules, such as the Pan-Cancer Detection and Digital Biomarker Panel, enable precise therapeutic targeting and faster clinical trials. Virchow2 is now also part of Paige's OpenPFM Suite on Hugging Face for research purposes.
Cancer of unknown primary (CUP) is difficult to diagnose. To address this, researchers created TORCH (Tumor Origin Differentiation using Cytological Histology), a deep-learning tool trained on images from 57K+ cases. TORCH identifies malignancy and predicts tumor origin in hydrothorax and ascites. Tested on both internal (12,799 cases) and external (14,538 cases) datasets, it achieved impressive accuracy for tumor origin localization, outperforming pathologists. Patients receiving treatment in line with TORCH's predictions had better overall outcomes but further validation is needed in randomized trials.
A team of Danish and Dutch researchers developed a Dual AI model that combines a diagnostic tool with a mammographic texture model to improve short- and long-term breast cancer risk assessment. The study, involving 119K+ Danish women with an average age of 59, used the Transpara diagnostic AI system and a deep learning texture model trained on 39K exams.
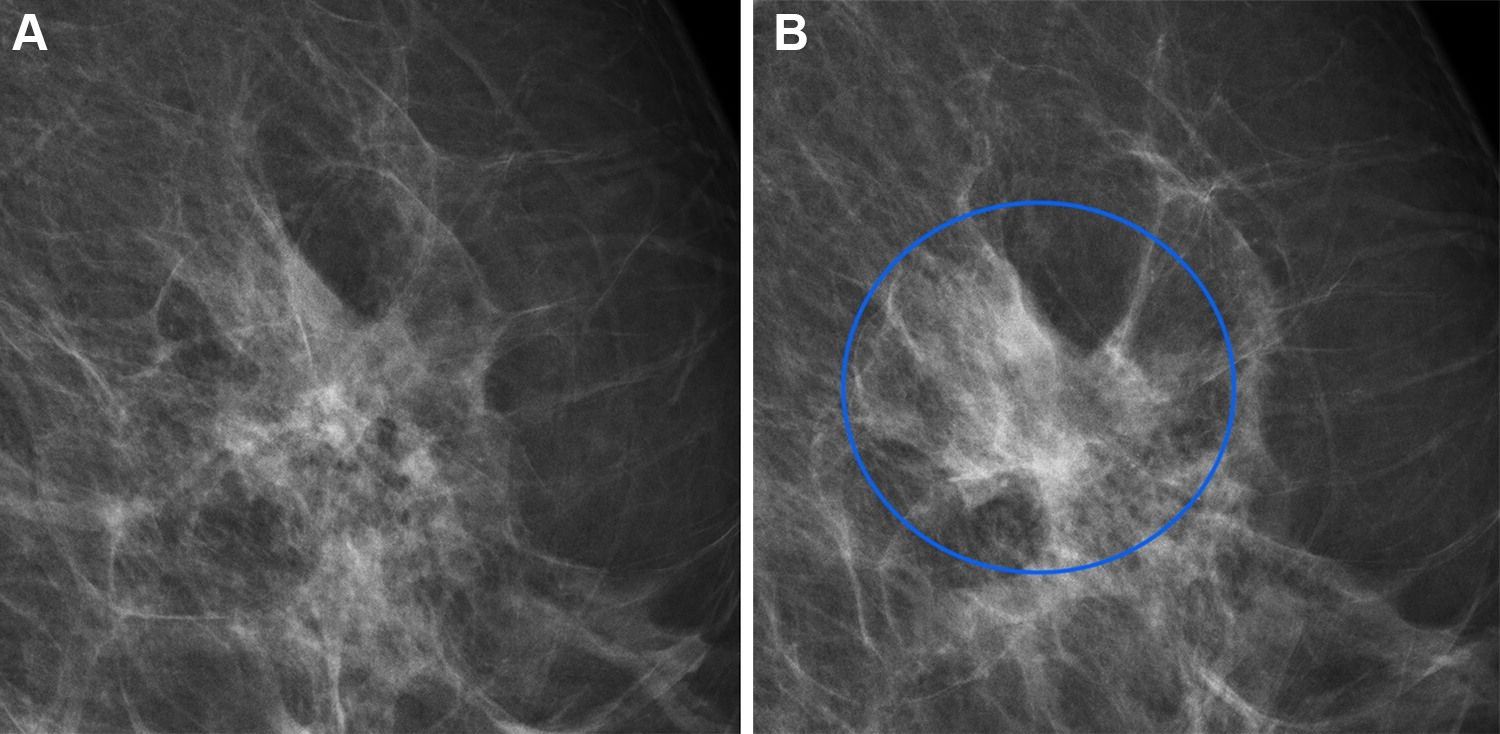
Full-field digital mammograms in a 60-year-old woman with an interval cancer. Source: https://shorturl.at/BUAY8
The findings showed that the combined model achieved a higher area under the curve (AUC) than using either model alone for short- and long-term cancers, identifying women at high risk. This AI-based approach allows risk assessment within seconds, minimizing the need for multiple tests and reducing strain on healthcare systems. Further research is planned to adapt the model for different mammographic devices and settings.
Using our model, risk can be assessed with the same performance as the clinical risk models but within seconds from screening and without introducing overhead in the clinic.
A Johns Hopkins-led team developed a method to generate huge datasets of artificial liver tumors on CT scans, enabling AI models to identify real tumors without human help. With only 200 annotated liver tumor scans available, this approach addresses the scarcity of data needed for AI training. The team used a four-step process to create hyperrealistic synthetic tumors, which even passed the Visual Turing Test, confusing medical professionals. The AI model trained on synthetic data only outperformed previous methods and matched models trained on real data. It can automatically generate examples of small tumors, improving early-stage cancer detection. This method can be applied to other organs, reducing the need for manual annotations by radiologists and accelerating AI-powered cancer detection.
Mayo Clinic researchers have developed an [AI model] (https://www.mayoclinic.org/medical-professionals/cancer/news/from-challenge-to-change-ais-leap-in-early-pancreatic-cancer-identification/mac-20558901), trained on over 3K CT scans, most of which came from external sources. The model achieved a 92% accuracy, correctly classifying 88% of cancer cases and 94% of controls. It also detected hidden cancers up to 475 days before clinical diagnosis in pre-diagnostic scans, with an 84% accuracy.
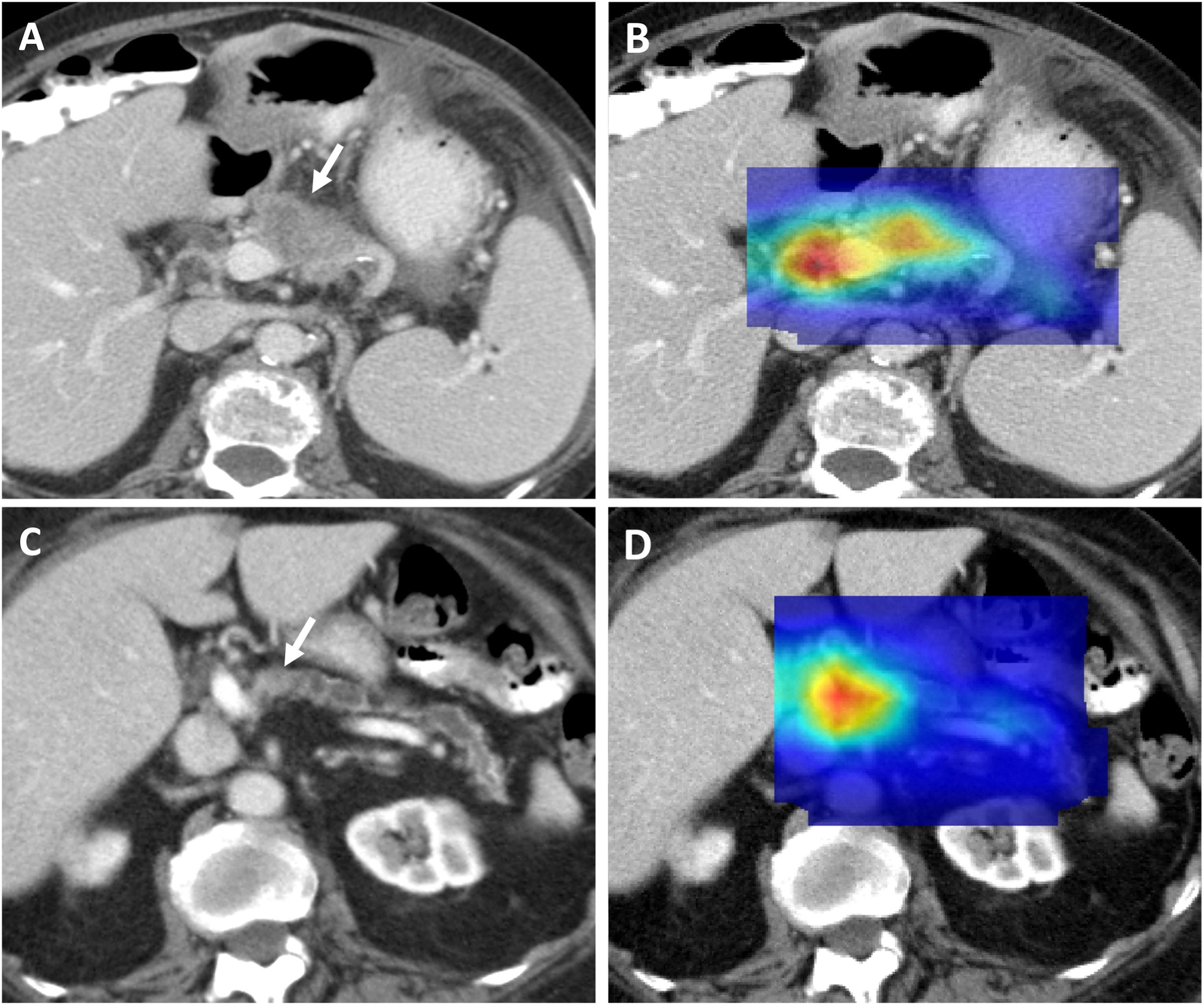
Axial portal-venous phase CT images show correctly classified malignant CTs and their corresponding heat maps. Source: https://shorturl.at/FTeiZ
This AI is fully autonomous, allowing integration into existing imaging workflows and assisting physicians by identifying subtle early cancer signs. Its reliable performance across diverse demographics shows its potential for real-world use. The team prepares for trials to further refine the approach.
The ability to identify cancer using AI-augmented standard-of-care imaging in seemingly normal pancreases at the pre-diagnostic stage is a major advancement in the field.
MIT and Dana-Farber Cancer Institute researchers developed a machine learning model, OncoNPC, to identify the origins of cancers of unknown primary (CUP). Using genetic data from about 400 genes commonly mutated in cancer, the model was trained on 30K patients with 22 known cancer types. Tested on 7K unseen tumors, it achieved 80% accuracy overall. Applied to 900 CUP tumors, it identified an additional 15% of patients who could have received targeted treatments had their cancer type been known. Patients who received treatments matching the model's predictions fared better than those who did not, highlighting its clinical value. Researchers plan to incorporate other data, such as pathology and radiology images, to enhance the model's predictive power.
Researchers at the Beckman Institute developed a new AI model for medical diagnostics that accurately identifies tumors and diseases in medical images while explaining its reasoning through visual maps. This flow allows doctors to understand and verify the model's decisions, improving trust and patient communication. The AI was trained on 20K+ images for 3 tasks: detecting early tumors in mammograms, identifying retinal abnormalities in OCT images, and diagnosing chest X-rays for cardiomegaly.
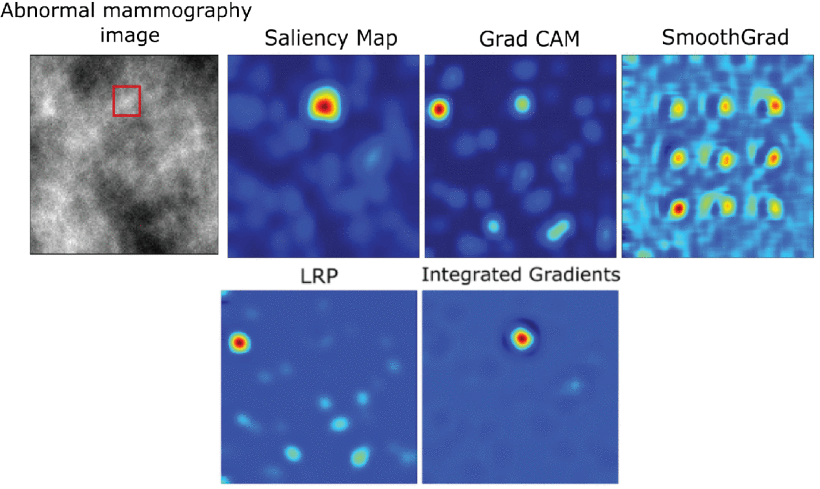
Heatmap interpretations generated by different post-hoc interpretability methods for the baseline CNN classifier. Source: https://shorturl.at/0oRS8
The model achieved high accuracy rates: 77.8% for mammograms, 99.1% for retinal OCT images, and 83% for chest X-rays. It matches existing AI models in performance but provides an "E-map" that visualizes its decision. This approach addresses the "black box" problem of AI, aiding doctors in double-checking its conclusions and offering clearer patient guidance. The researchers aim to expand this model to detect anomalies throughout the body.
The idea is to help catch cancer and disease in its earliest stages -; like an X on a map -; and understand how the decision was made. Our model will help streamline that process and make it easier on doctors and patients alike.
The unique AI model for skin cancer risk assessment identifies features invisible to the human eye. Developed by dermatologists and AI experts at Erasmus MC researchers, it was trained using over 2.8K facial photos, each taken under the same conditions, with known skin cancer outcomes. The algorithm extracts 200 features from these photos to predict skin cancer risk more accurately than traditional methods like skin examinations, questionnaires, or genetic research.
The model employs explainable AI, allowing researchers to visualize and understand what features the algorithm uses for its predictions, such as wrinkles, and other less obvious characteristics. In the future, the model could aid in skin cancer prevention and awareness. While not yet clinically usable, researchers aim to further test the model and encourage collaboration by publicly sharing the model for research purposes.
Limitations & Challenges
AI holds enormous potential in oncology, particularly in improving cancer imaging and diagnostics. Yet, while many AI tools have shown promise in early tests, their practical application is still in the early stages. There are quite a few limitations and challenges that we summarize below and that should and can be addresses so that we move forward faster towards cancerless future.
- Large Data Requirements: AI models need thousands to tens of thousands of annotated images for training and validation, which is often difficult to obtain, especially in oncology. The creatvie solution could lie in using diverse sources, such as social media (e.g., Twitter) where pathologists share complex cases for varied data.
- Learning Spurious Correlations: Models can mistakenly associate technical artifacts, such as staining techniques, with patient outcomes, leading to incorrect generalizations. To address this, researchers may invest in ensuring generalizability across hospitals and conditions, using heterogeneous data sources to minimize these spurious correlations.
- Bias in Training Data: If training data predominantly features one ethnicity, sex, or region, the model may not perform accurately for underrepresented groups. The solution is clear — include diverse data in all stages — training, evaluation, and post-deployment monitoring.
- Lack of Explainability (Black Box Problem): AI often struggles to explain how it arrived at a decision, making it hard for clinicians to trust its outputs. Developing AI models (one example mentioned above) that generate visual maps or identify key variables contributing to predictions can increase transparency and build clinician confidence. Also, incorporating alerts when models might be operating outside their trained data scope would adda a layer of reliability.
- Physician Hesitation and Automation Bias: Clinicians may hesitate to use AI due to unfamiliarity or lack of explainability. Conversely, overreliance on AI (automation bias) can lead to misinformed medical decisions. Building human-in-the-loop models allows for real-time clinician feedback, ensuring AI aids decision-making rather than replacing human judgment.
- Regulatory and Ethical Barriers: Privacy rules and the lack of standardized data collection methods make it difficult to pool high-quality datasets. Additionally, accountability concerns exist regarding who is responsible when AI predictions are incorrect. A clear regulatory oversight, ensuring patient privacy, and standardizing data collection may be needed to facilitate the responsible use of AI. Creating workflows where AI complements clinical decisions can help balance innovation with ethical considerations.
- Limited Real-World Application: Despite AI's potential, adoption is limited due to logistical issues in creating workflows and concerns about flawed decision-making. That said, with more tools, education and regulation, we expect AI to be increasingly integrated into workflows to be used continuously in clinical practice.
Have an AI project that needs help?
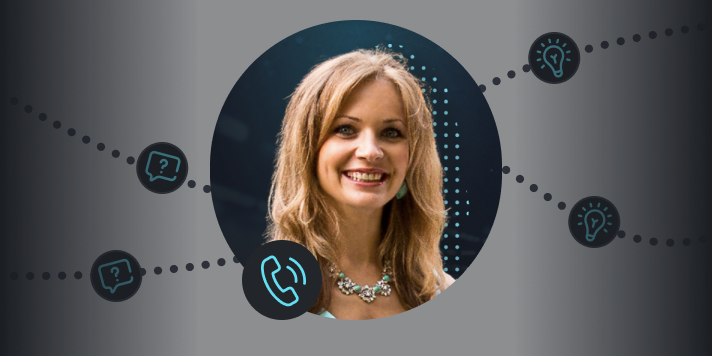
Talk to Yuliya. She will make sure that all is covered. Don't waste time on googling - get all answers from relevant expert in under one hour.