Ali H. Raza, CEO of ThroughPut.ai, on the Next-Gen Supply Chain Management
How ThroughPut.ai Reshapes Supply Chain Dynamics with AI and Manufacturing Integration
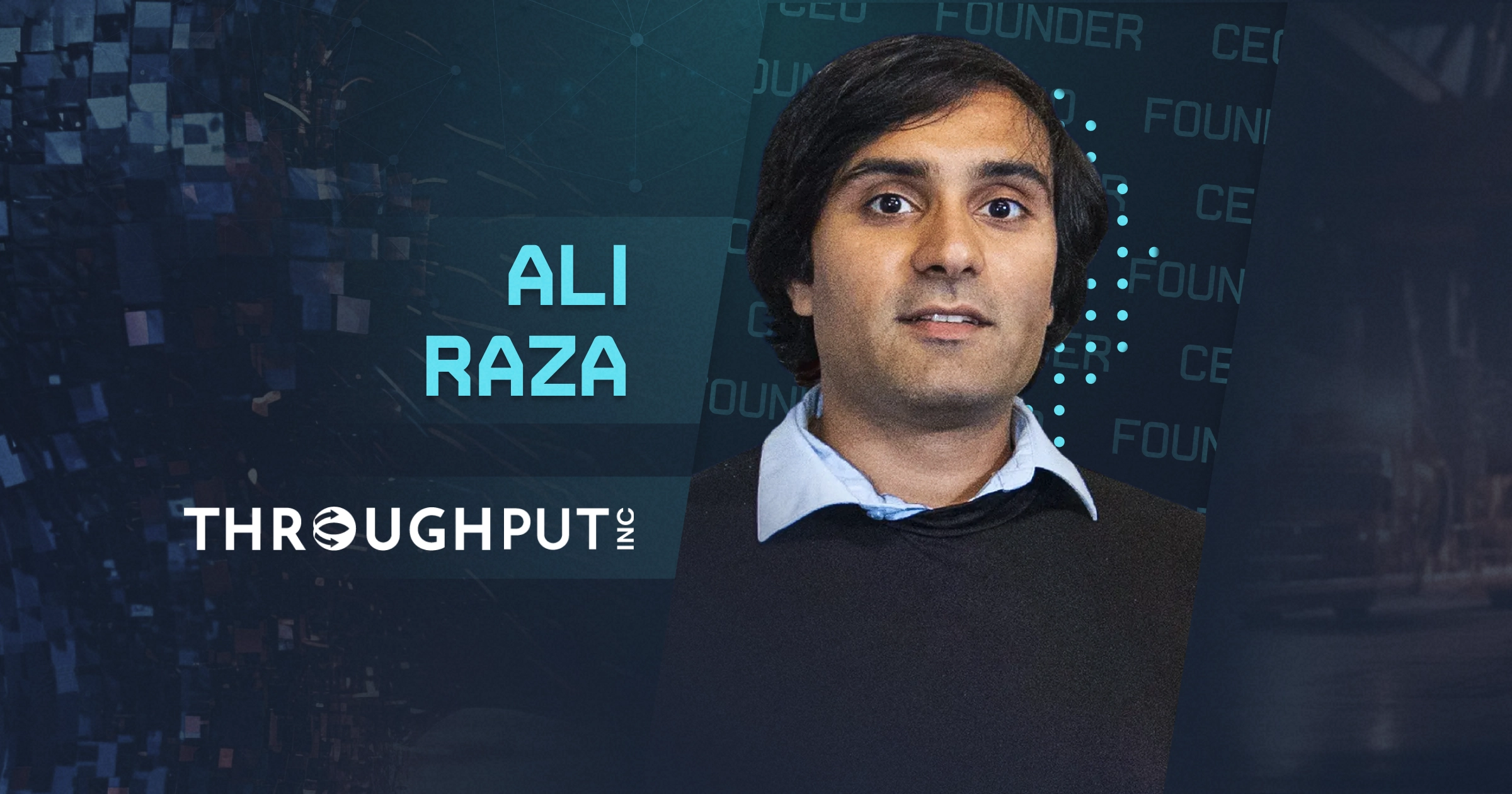
Ali H. Raza is the Founder and CEO of ThroughPut.ai, a supply chain intervention software provider that empowers owners & CXOs to generate cash from their existing operations by accelerating supply chain decisions. The platform provides the fastest time-to-value from existing supply chain-related data sets. ThroughPut raised $8.5M in Private Funding from early investors in Robinhood, NeXT, Project44, and leading industrial executives.
Yuliya Sychikova (YS): Ali, how do you define the vision for ThroughPut?
Ali H. Raza (AR): ThroughPut.ai will be the most influential technology company for industrial boards, CEOs, and shareholders to turn around their business performance starting with the supply chain.
(YS): Both of us went to the University of Pennsylvania. In which ways did earning BS and MS degrees in Chemical Biomolecular Engineering inspire and help you establish ThroughPut.ai?
(AR): I first discovered process flow bottlenecks in my senior process design class (teaching the class the following year as a graduate student), and how the problem scaled to real-life scenarios like logistics and manufacturing. Being taught the financial impact of such problems is something Penn/Wharton gets deeper into than most schools. This is why ThroughPut.ai links the supply chain data in order to impact the Earnings Per Share of a company.
Today, ThroughPut.ai holds patents on Industrial Bottleneck Detection that companies like TSMC and Oppo leverage to resolve their “throughput” and “inventory” problems.
After graduating, I worked in field operations across 8 countries to improve my understanding of logistics & manufacturing, with my last role being the geomarket manager of Yemen in 2015 when the Houthi government took over. What you are seeing in the Red Sea today, my operations teams dealt with at a smaller scale back then.
(YS): Your solution has a helpful ability to incorporate manufacturing into the analysis. Why is it important?
(AR): Until COVID, American manufacturing was very much on the decline, to the point where a lot of supply chain planning technologies were treating ”manufacturing plants” as “warehouses” in their models. However, unless this involves remanufacturing, you cannot assume that the initial product is the same as the end product. With manufacturing, the goods change and the flow is often disrupted, and this needs to be handled effectively.
As we are discovering with semiconductor supply chains, if you don’t know your “true capacity” to make stuff, then how can you plan an entire supply chain around it successfully, let alone your next big capacity buy? Demand, supply, and capacity have to all be factored in. These sorts of systems were absent when I was in field operations, and for the most part, are absent today for operation management decision making. There are plenty of tools for Operations Researchers and Data Scientists, but not the actual end user responsible for the Net Income Statement.
Today, when quadrants are released, you see companies on the Supply Chain & Demand maps and then companies on Industrial Manufacturing/4.0 maps, but none of them really cross over. They are part of the same value chain, and that is why we determined we need to do both. Capacity planning is one of the top asks from our inbound supply chain leads.
We are one of the first companies to factor in lead times and changing demand over bottlenecks in the value chain. Our logic visualizes inventory stockpiles, and rebuilds trust with suppliers based on true lead times vs. historical failures.
(YS): One of the main things about a successful product is the ability to tell a story using the software and not just display the data. How does your platform allow you to do that?
(AR): We have the fastest turnaround time for a material management data set. This bypasses the traditional 1-2 year pilot to scope/seek value, by delivering the value in as early as a week to the direct end beneficiaries in sales & operations. We establish ground realities fast to the top, as well as available actions to take to generate cash in any business environment (boom/bust/cruise).
With exports related to material flow data, we are able to quickly show the board 3 opportunities to generate more cash:
-
How to match global supply to local demand changes. This is to say that products that sell in Donetsk might not necessarily sell in Kyiv due to a variety of factors (weather, demographics, unplanned disruptions). Segmentation helps drive contribution margins.
-
How much inventory to carry. We are one of the first companies to factor in lead times and changing demand over bottlenecks in the value chain. Our logic visualizes inventory stockpiles, and rebuilds trust with suppliers based on true lead times vs. historical failures. This eases the working capital stress, and relaxes the overall supply chain to turnover more inventory. If sales are picking up, the system can recommend larger quantity orders or holding on to more buffers.
-
How to get more out of existing capacity. Rebalancing flow around bottlenecks, especially of individual products, “creates capacity”. Oftentime, this means delaying CAPEX decisions, which helps CFOs invest elsewhere for the year.
(YS): ThroughPut.ai is an AI-driven product. What role does AI play in your product?
(AR): It’s important to note that supply chain & logistics as industries are probably at Level 0 or Level 1 of the AGI spectrum. In other words, most cases still require human-in-the-loop, but when individual use cases are properly defined (aka “Narrow AI”), AI can be appropriately incorporated to accelerate certain decisions/sorting.
Today, AI is used in our software to predict next day prices and adjust allocation volumes for individual customers. Dynamic segmentation and reprioritizing customers on an on-going basis unlocks more cash flow.
Additionally, ML can be used to optimize individual and multiple factory schedules. If you have incoming data on changing supply, demand, and capacity, you can dynamically adjust inventory levels.
(YS): How did you train your algorithms?
(AR): ThroughPut’s vision is to be the most influential technology company in the boardroom. As mentioned before, this requires us to build trust around receiving internal supply chain data and tying it with external data sources (prices, election cycles, weather, and many others) to unlock real cash. These inputs power our algorithms, as does human-in-the-loop feedback.
ThroughPut’s vision is to be the most influential technology company in the boardroom. This requires us to build trust around receiving internal supply chain data and tying it with external data sources (prices, election cycles, weather, and many others) to unlock real cash.
(YS): Could you please provide examples of how ThroughPut.ai leverages AI to make real-time corrective decisions?
(AR): Within maintenance departments, ThroughPut looks ahead at planned and unplanned maintenance indicators, as well as looking back at lead times, to readjust required inventory buffers to ensure uptime without additional working capital costs. An example customer is the NYC Subway, where we have sourced parts internally by creating faster flow, and thus virtual inventory stock.
Within farming, ThroughPut monitors the price of various produce, and recommends the next day item's price (as high as 93% accuracy) and goes further by allocating the right volume to the right customer. This multidimensional reorganization of price, volume, and target customer (especially for variable contracts) would be difficult without technological assistance.
Within military applications, ThroughPut does what is known as predictive kitting. It’s similar to how Amazon knows how you might need coffee filters when you order coffee, but instead, for rescue plane parts.
(YS): AI can do so much in logistics, hypothetically: from managing inventory and fleet utilization to autonomous driving and reducing carbon emissions. Where do you see ThroughPut.ai making the most impact for your clients?
(AR): ThroughPut.ai provides an Executive Management System that brings focus to bottlenecks as “the problems” to solve first. The biggest impact of ThroughPut.ai is quickly finding these bottlenecks (or the company’s overall constraint), and showing how optimization around a few points can prevent costly capital expenditures. For example, a new semiconductor fabrication unit could cost $10BN and many years to build out. Even if AI brings a 1% improvement in output by “scheduling better”, that’s hundreds of millions of dollars that can be realized today by quick fixes. NVIDIA’s manufacturing division needs that today, and TSMC manufacturing division already cites our work.
It is important to note that this level of impact may not require AI to start, but appropriately presented data visualizations can do the initial work. Sometimes, customers have to be gradually stepped up to AI.
(YS): Do you plan on incorporating the power of LLMs? If so, in what way? What is next on your AI product roadmap?
(AR): For customers, no. While we use LLMs internally to improve our workflows, we still live in an environment where data is messy and requires a lot of mapping and cleaning. LLMs can potentially help with that effort, but that is not our core business. I am seeing LLM Supply Chain startups pop up, but the big limitation is data access and cleanliness.
Our core business is to help boards understand their “true capacity to fulfill” at any given time and in the future, and there are AI capabilities on our roadmap tied to that.
The current bottlenecks in the domain are open product competition, and access to top decision makers. The Logistic Tech industry would benefit from adopting a problem solving-first approach rather than a marketing-first approach.
(YS): What are your thoughts on the future of AI in Logistics and current roadblocks?
(AR): Since logistics is a global industry, it’s best to highlight the geomarkets ThroughPut.ai operates in, and where they currently stand. While this may upset many domestic logistics advocates, North America lags Asia by 10 years and Europe by 5 years. This doesn’t have as much to do with the local talent, but the data readiness/fragmentation of the industrial ecosystem. India, LATAM, and the Middle East are good product testing environments due to easier owner access, and thus data access.
The current bottlenecks in the domain are open product competition, and access to top decision makers. The Logistic Tech industry would benefit from adopting a problem solving-first approach rather than a marketing-first approach.
Fundamentally, logistics optimization is a math problem, and the math doesn’t really change (nor does transportation physics). The technologies involved help accelerate, segment, and scale as they improve. Considering Small Businesses power 44% of the Economy (Source: SBA 2019), Logistics AI has not yet achieved the unit economics to make Narrow AI accessible to all.
For the most part, CEOs & CFOs still need capability demonstrations to really buy in to approve CIO and Chief Supply Chain/Logistics Officer requests. In physical industries, it’s typically more challenging to sell software as the end user is seeking tangible productivity validation.
That’s where ThroughPut.ai comes in.