AI in Manufacturing: Precision and Efficiency Redefined
How Smart Tech Drives Quality, Efficiency, and Sustainability.
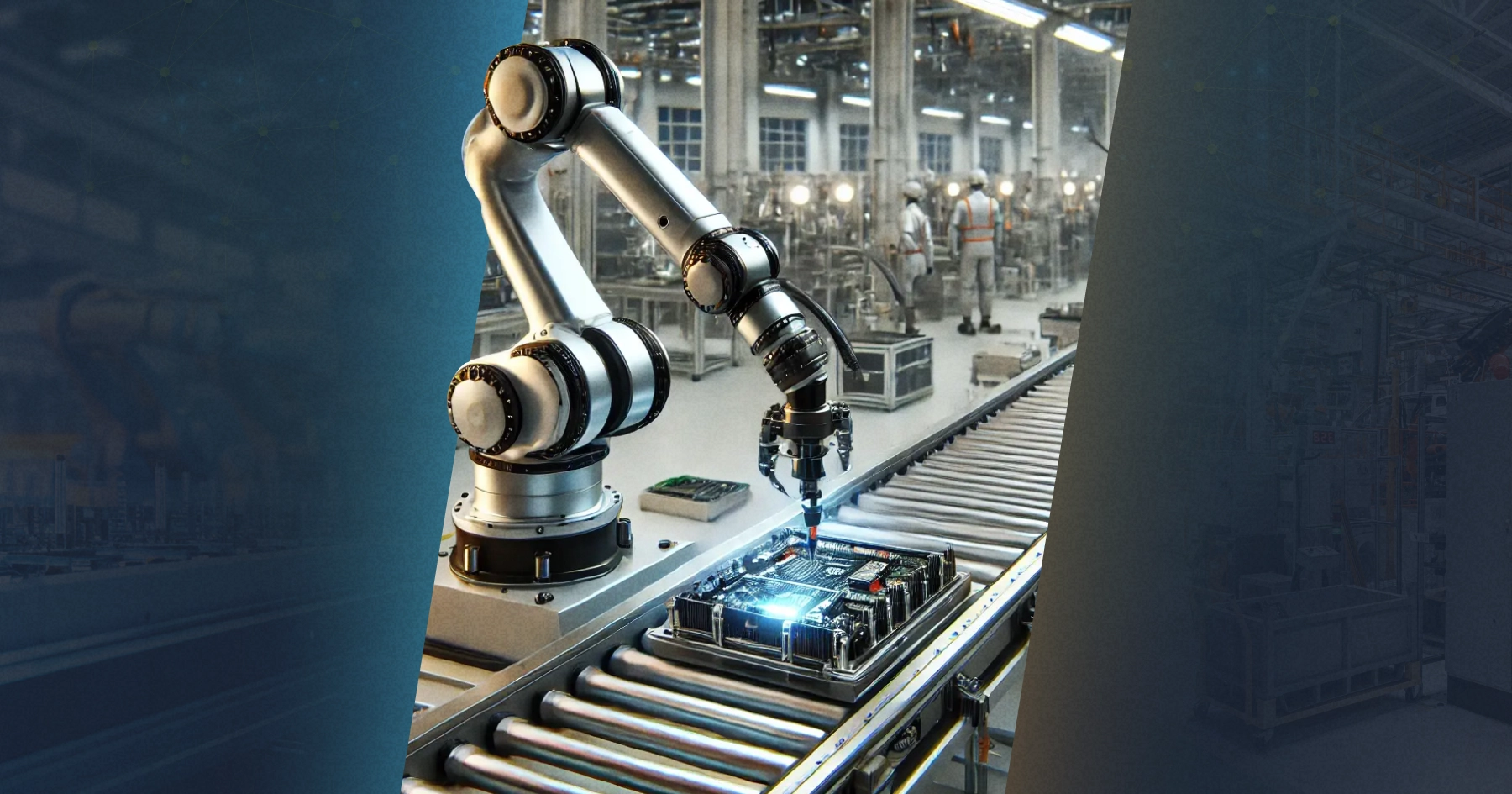
The manufacturing world has just entered a new era, which is bound to be "smarter" with the rise of AI. Improving automation, efficiency, and cost reduction makes AI stand out as an essential factor in shaping the future of the industry. As companies worldwide are adopting AI-driven solutions rapidly, smart manufacturing (SM) has quickly emerged as the industry standard.
Let’s dive into SM processes that are powered by advanced technologies.
Fortune Business Insights predict the global smart manufacturing market to grow to $658.41B by 2028. The market size will increase from $310.9B in 2023 to $754.1B by 2030.
Source Fortune Business Insights
Enhancing Automation and Productivity
AI-powered automation has transformed manufacturing into barely manned operations. From product assembling to quality checking, AI-powered robots can handle massive operations. Such intelligent systems can carry out work quicker and more accurately, reducing the margin for error. Also, AI's capability to process bulks of real-time data enables machines to quickly act on changes in production demand, optimizing output and reducing potential downtime.
On the manufacturing front, gen AI can unlock untapped productivity during production, leveraging root cause analysis to predict failures and reduce defects, and draft easy-to-follow dynamic work instructions. It can also augment operator stations by offering live, AI-supported troubleshooting and operating guidelines.
A good example is Siemens’s infusion of AI-enhanced automation into the Amberg Electronics Plant in Germany, often termed a "factory of the future." Churning out more than 15 million programmable logic controllers annually, this factory has minimal human interference as it automated at least 75% of the whole production process - from handling raw materials to testing the finished product. The AI systems is to continuously optimize the production schedules, finding the bottlenecks, and making real-time adjustments toward near-zero defect rates.
Tesla produces its EVs at gigafactories, where a great part of the process is automated by AI-powered robots. These kinds of robots easily execute complex operations such as welding, painting, and assembling car parts with very little human intervention. Tesla's robust AI algorithms allow such robots to work quickly, lowering production costs and meeting rapidly growing demand.
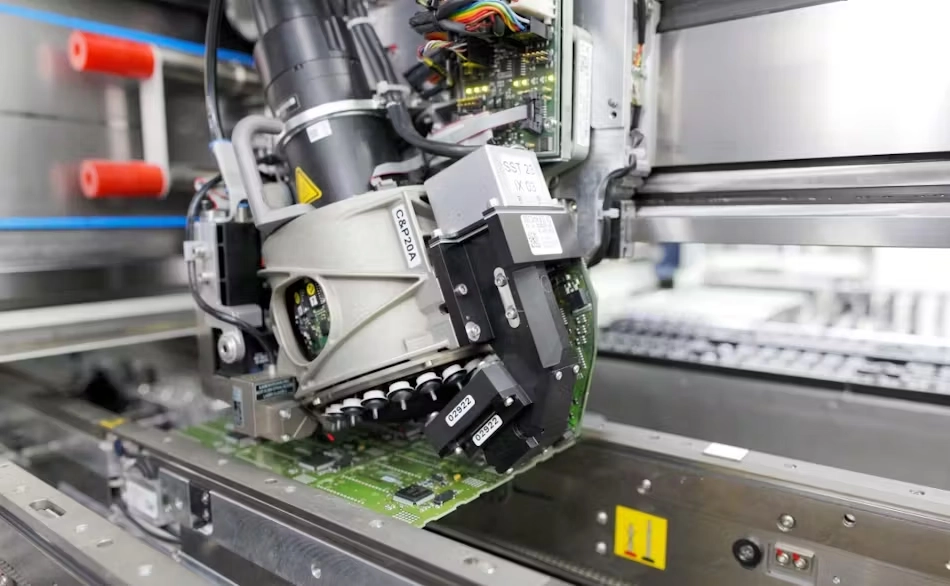
At the Amberg facility, AI assesses the likelihood of errors in assembling printed circuit boards
Improving Quality Control and Defect Detection
AI probably contributes most to smart manufacturing in quality control. Traditional quality checks often involve human inspection, which is prone to errors, especially at large production volumes. On the other hand, AI computer vision systems can perform product checks faster and more precisely.
For example, companies like BMW employ AI computer vision for vehicle inspection directly from the assembly line. The system processes frames in real time using high-resolution imagery to find defects that range from imperfect paint to minute scratches to misaligned parts. As AI gets smarter over time, its detection rates improve ensuring only quality vehicles enter the market.
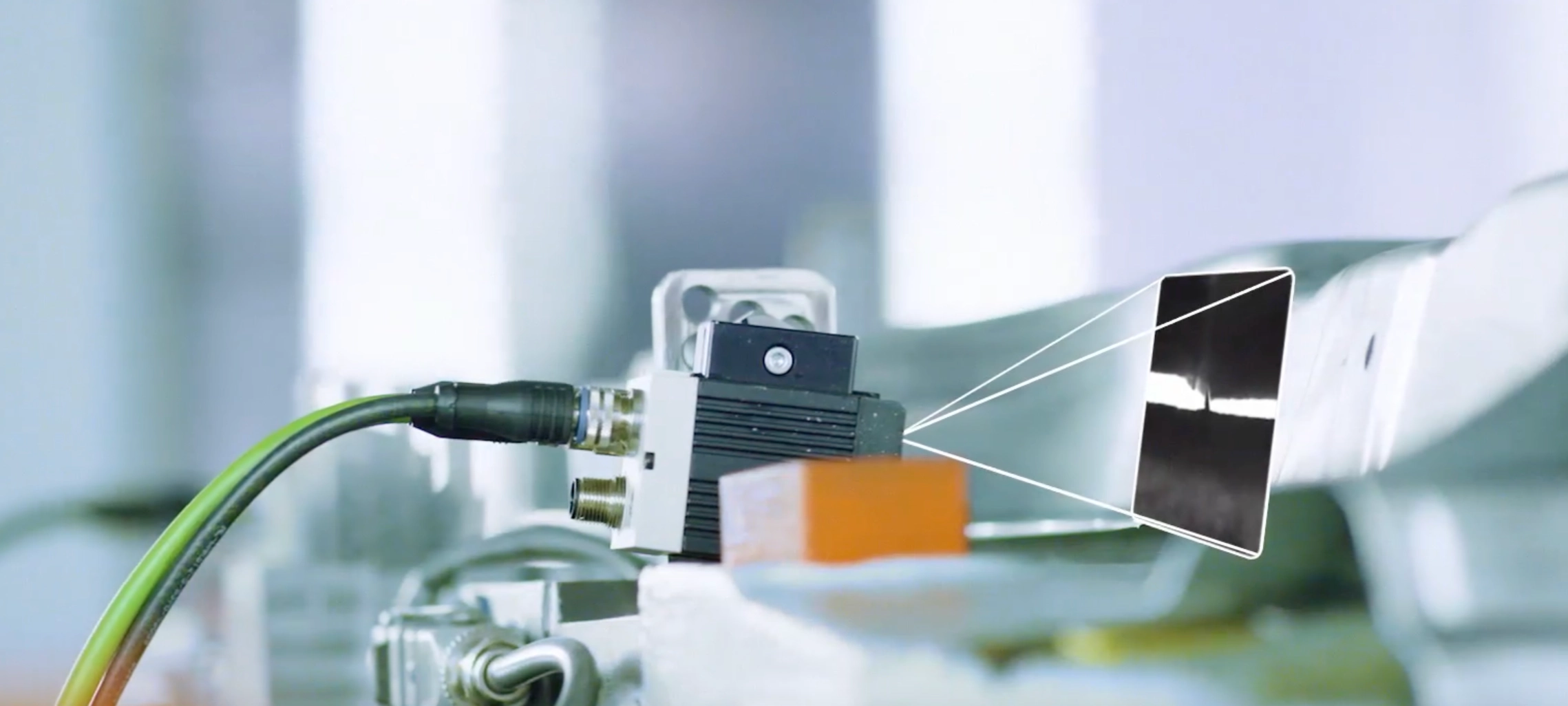
AI eliminates pseudo-defects at crack control, BMW Group Press Plant Dingolfing
Hyundai Transys is enhancing its production quality through its AI system, Transys Advanced Data Analytics (TADA). This in-house-developed digital platform leverages AI to improve decision-making and employee digital skills. Key components include the TADA Smart Solution, which uses deep learning to boost defect inspection accuracy from 93% to 99.9% by identifying flaws during manufacturing that human inspectors might miss, and the TADA Edge Solution, a cost-effective AI inspection tool that allows non-experts to create deep learning models on-site.
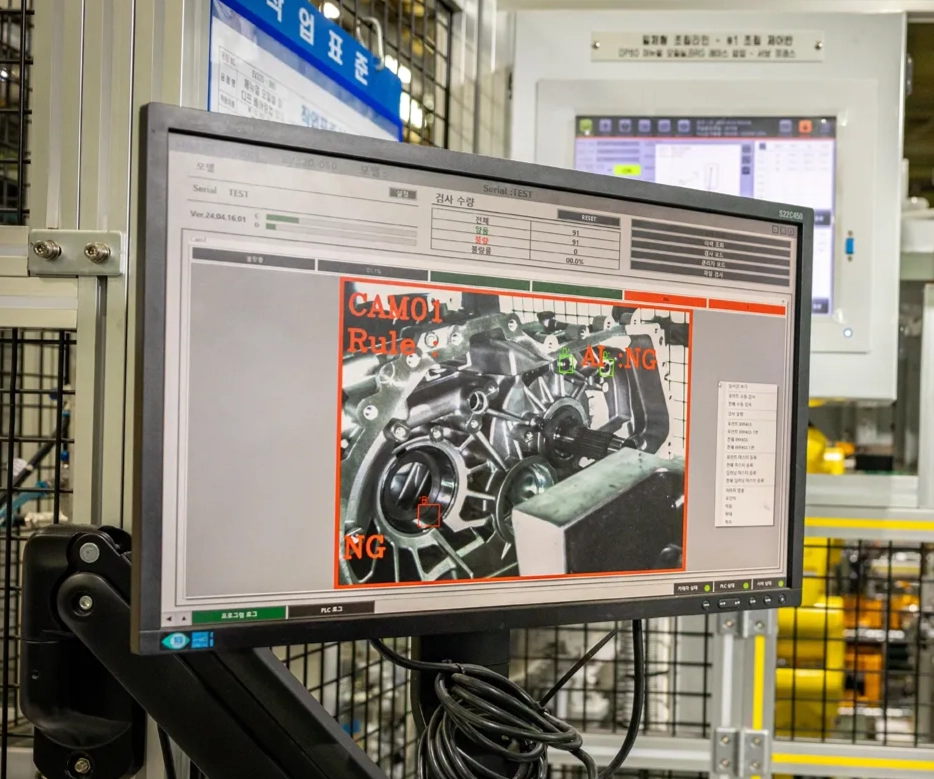
TADA Smart Solution detects bubbles or damage in parts during scanning.
These AI systems could also be tailored to specific industries. For instance, in the semiconductor industry where precision is everything giants like Intel use AI-powered inspection systems to spot microscopic defects on wafers and chips. This reduces the risk of defective products hitting the supply chain, avoiding costly recalls, and preserving consumer confidence.
Predicting Maintenance
Predictive Maintenance (PdM) uses machine learning on sensor data to detect machine failures early, reducing unplanned downtimes and resource consumption.
Caterpillar, one of the leading construction and mining equipment manufacturers, uses AI for predictive maintenance in tracking heavy machinery service and maintenance. Their system, known as Cat Connect, monitors temperature, pressure, and vibration for various parts of machinery. With these details, AI algorithms can predict potential failures and schedule maintenance preemptively. This approach minimizes manufacturing and service facility downtime for Caterpillar, improving the equipment reliability.
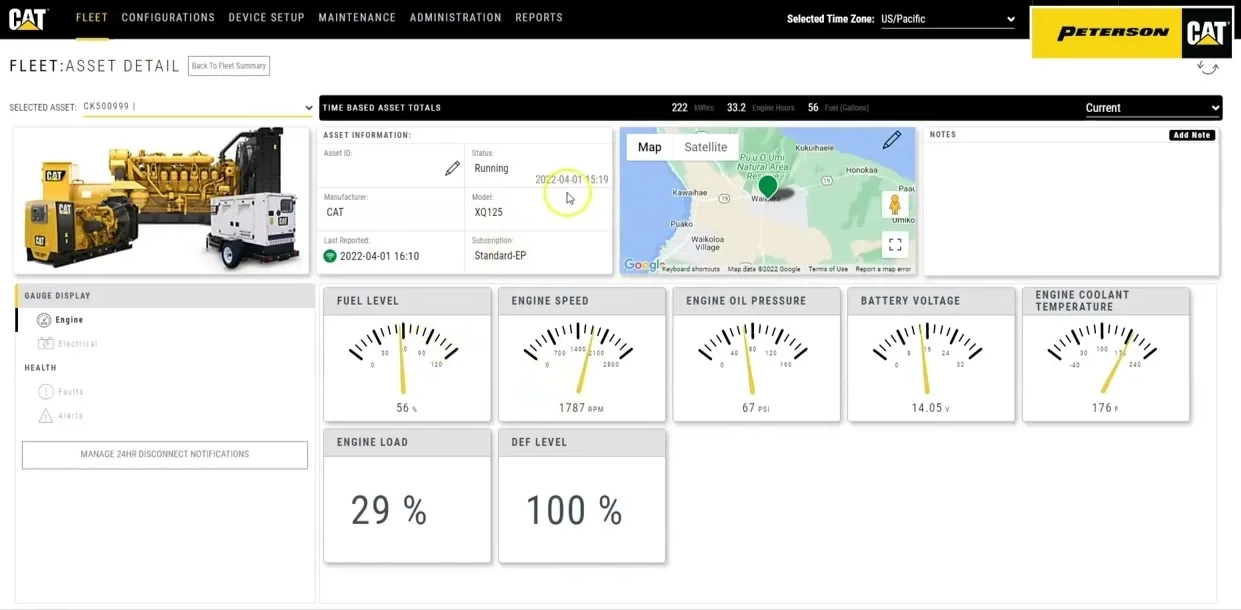
CAT Connect Dashboard
Precision Manufacturing
Precision manufacturing involves creating highly accurate and workable components from a design by removing pieces of material with tools like computer numerical control (CNC) machines until the final shape is achieved. Artificial intelligence is a growing part of precision manufacturing, enabling real-time monitoring, quality control, and optimization of processes right on the production floor.
Leading manufacturers are integrating AI-powered systems within their value chains to realize efficiency and quality. For example, Intel leverages AI in semiconductor fabrication to detect minute aberrations that can affect chip yields.
In aerospace, Boeing deploys AI-driven robotics systems for drilling and riveting at high precision, accuracy, and speed with minimal human intervention to ensure structural integrity.
Tesla uses real-time data analysis in the manufacturing of its batteries, enforcing the most stringent quality control in such critical factors as electrode coating thickness and cell assembly.
Similarly, Siemens leverages its MindSphere platform to decrease PLC (programmable logic controller) defects using machine learning to identify anomalies in manufacturing parameters.
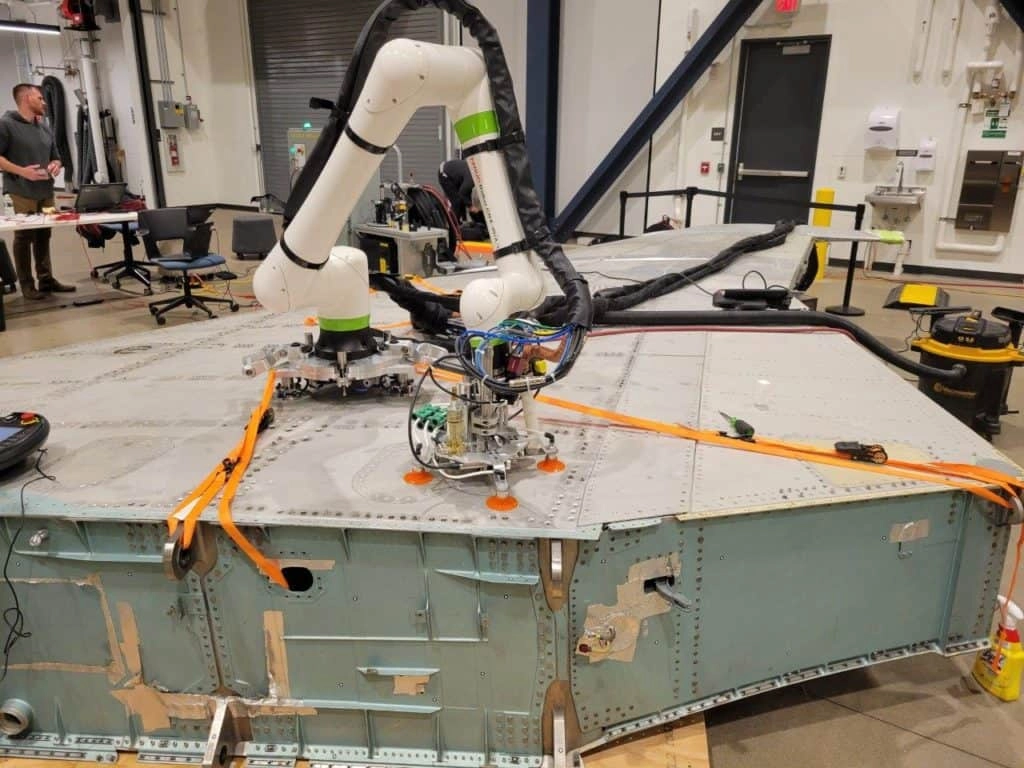
Boeing deploys AI-driven robotics systems for drilling and riveting at high precision
Indeed, AI-driven precision manufacturing has massively reduced waste, production downtime, and overall production costs across many industries, including electronics, aerospace, automotive, and consumer goods. These advancements also support sustainability by minimizing material and energy waste. As AI’s capabilities thicken, its role in precision manufacturing will deliver even greater accuracy and efficiency, setting new standards for quality and innovation globally.
Optimizing Supply Chain Management
AI also performs stably in the optimization of supply chains, the backbone of any manufacturing process. By applying AI algorithms to supply chain data, companies can gain insights into demand trends, improve inventory management, and anticipate potential disruptions before they occur.
AI is going to redefine the meaning of connected manufacturing and supply chain operations. First movers have the greatest potential to capture the value available and improve productivity.
At Amazon, AI optimizes product movements across the global fulfillment centers by predicting the best delivery routes and managing the inventory needed to anticipate customer demand. This real-time optimization saves money and guarantees improved customer satisfaction.
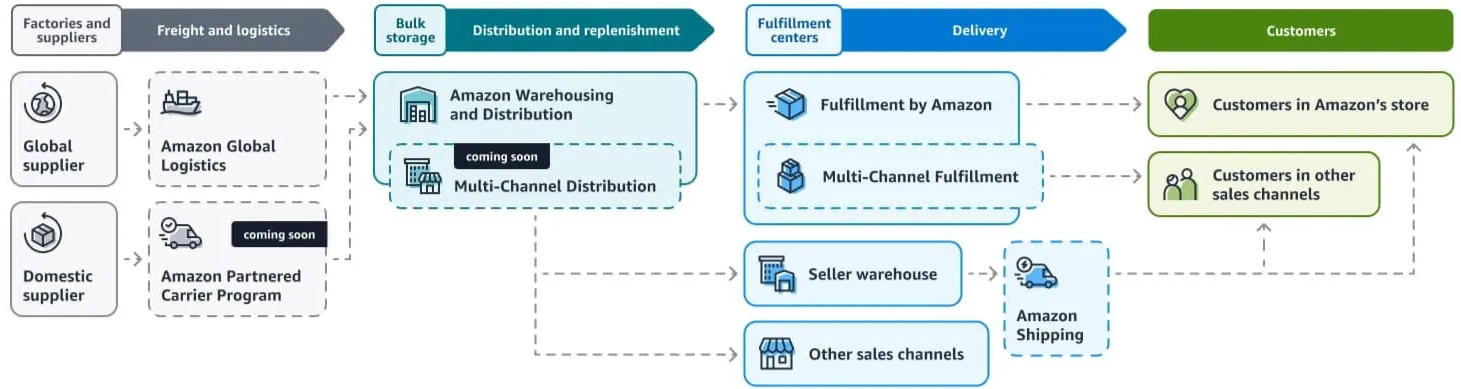
Supply Chain by Amazon
Unilever's tapping of AI improves their demand forecast and supply planning. Their "Digital Twins" program models the entire supply chain to simulate production and delivery processes. The result is a real-time, data-driven view of the supply chain operations. This has allowed Unilever to shave the inventory costs, reduce waste, and make better production scheduling decisions to meet consumer demand every time, including peak periods.
This is all helping us to bring new innovations to market at a pace previously unimaginable. We’re responding to consumer needs, faster and better than ever before.
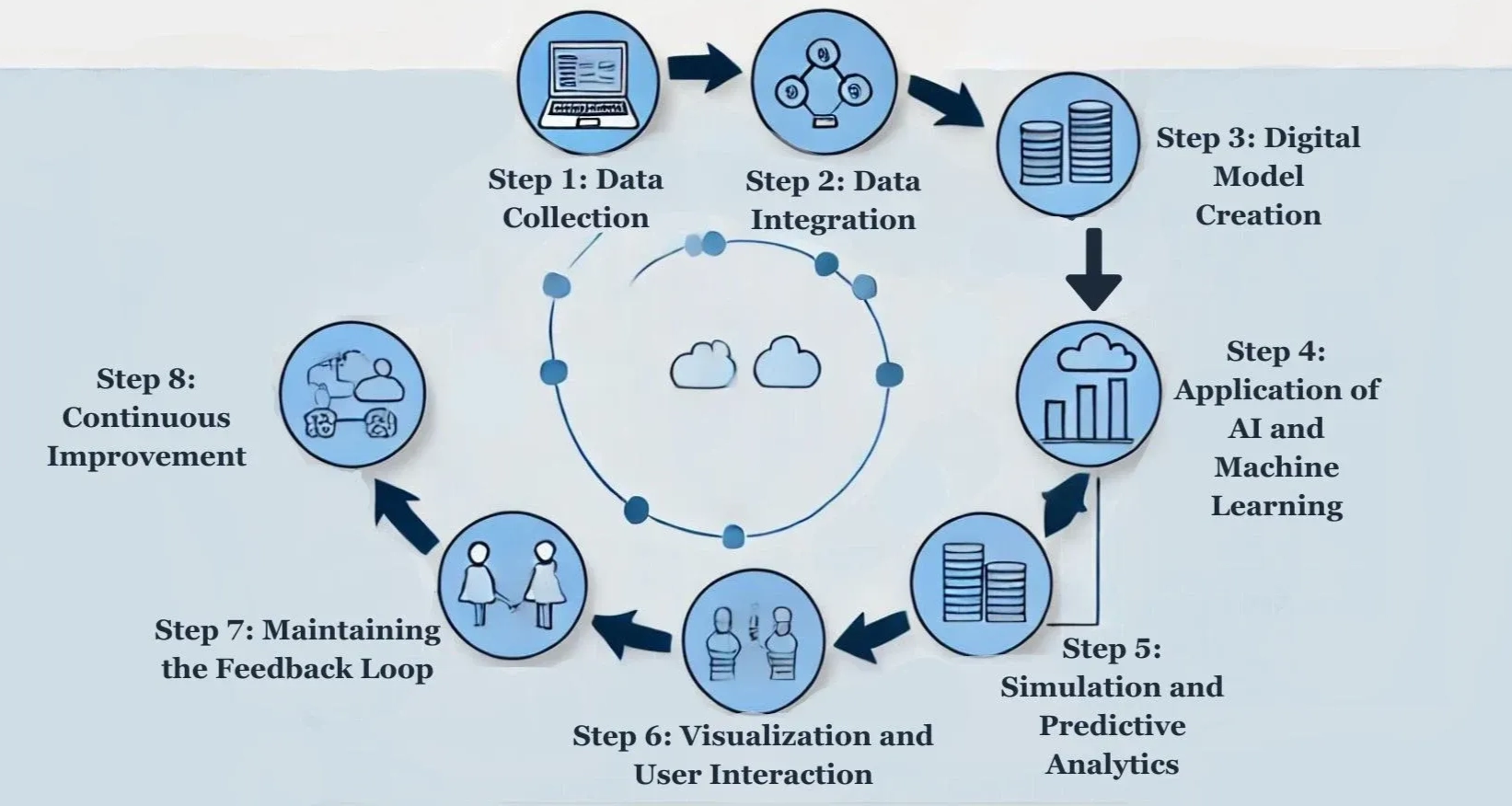
Illustration of the Digital Twin step-by-step process
These examples show how various companies leverage AI for resilient, responsive, and efficient supply chains. With smart technologies, companies can be better prepared to foresee and overcome any challenge, while minimizing costs and meeting rising consumer demand.
Supporting Sustainability Efforts
Most manufacturing companies today aim to make their operations greener. AI’s ability to analyze energy data at each production stage enables optimized energy use. Manufacturers can minimize their carbon footprint and reduce the cost of energy by pinpointing inefficiencies.
A prominent example is Schneider Electric, which implements AI-powered software to optimize its energy management at every factory. Using data obtained from sensors across the facilities, Schneider Electric can identify where energy use can be reduced, further helping in achieving environmental sustainability. Through this, the company was able to reach its sustainability goals of reducing CO2 emissions and promoting the use of renewable energy.
When global steel producer Gerdau incorporated AI to streamline its use of ferroalloys, it reduced its alloy costs by $3 per ton while improving its Scope 2 (indirect energy greenhouse gas emissions) and 3 (other indirect greenhouse gas emissions for units in Brazil, US and Canada) footprints by reducing the overall volume of alloy additives it needed to purchase and deliver each year.
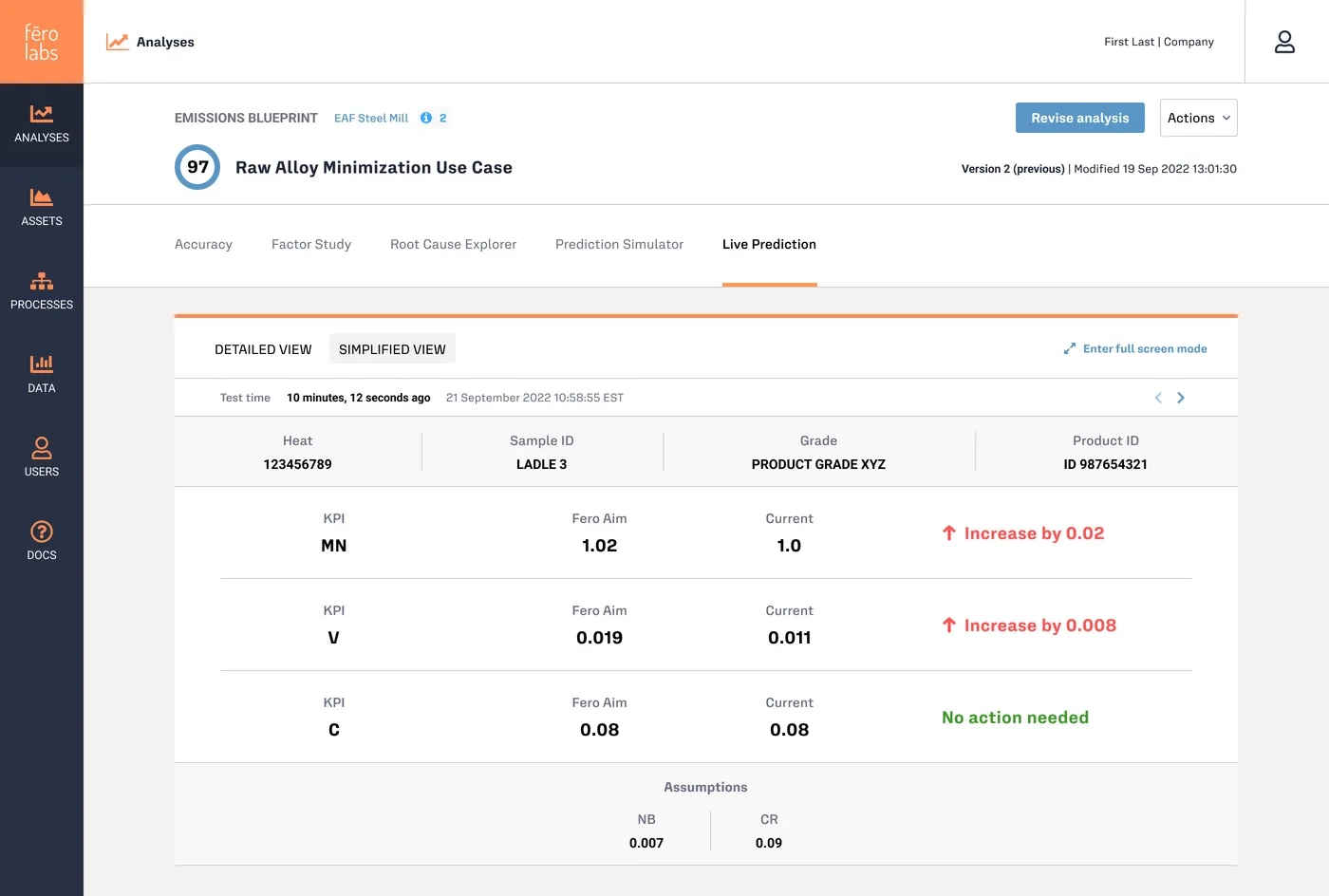
Gerdau minimizes Raw Alloy usage with the help of Fero Labs software
What’s next?
AI for smart manufacturing will become increasingly transformational, driving further efficiencies, precision, and agility across industries. Some examples of its future role include:
- Autonomous manufacturing with automatic adjustment of systems in a closed-loop manner.
- Generative design rapidly optimizing products for quicker delivery and supporting on-demand customization.
- AI-driven quality control, predictive maintenance, and digital twins to minimize defects and downtime, offering real-time insights for continuous improvement.
- Collaborative robots (CoBots) that seamlessly work with human workers to extend productivity and increase safety.
- Sustainability practices will further benefit from smart technologies in waste reduction and resource optimization.
- AI-powered supply chain forecasting and Edge AI will, in turn, enable responsiveness to disruption and decentralized decision-making.
- Enhanced cybersecurity, powered by advanced intelligence, secures manufacturing systems against the growing cyber threats due to increased digitalization.
These contributions place AI at the heart of creating a more resilient, high-quality, and sustainable manufacturing ecosystem in the future.
Risks & Challenges
While adoption of AI in smart manufacturing offers so many enticing benefits, at the same time it introducing significant risks. Some of them include high implementation costs, possible cybersecurity vulnerabilities, job displacement and dependence on data quality.
According to ”Harnessing generative AI in manufacturing and supply chains” by McKinsey, these challenges might inlcude:
- Risk of data leakage in AI model inputs
- Potential compromise of intellectual property (IP)
- Exposure to liability under regulations like International Traffic in Arms Regulations (ITAR) or Health Insurance Portability and Accountability Act (HIPAA)
- Inherent biases in models that may unduly amplify specific data segments, affecting result reliability
- Emphasis on maintaining human responsibility as AI models require active, conscientious oversight by human operators
There are ethical and regulatory concerns arising about the accountability of AI, especially in case of “black box” models that are difficult to interpret for decision-making purposes. Moreover, the integration of AI into the existing infrastructure could also be problematic due to the older equipment, complicating smooth operations.
Conclusion
Artificial Intelligence has become one of the major drivers of the revolution in smart manufacturing, opening up new ways of creation, assembly, and distribution. Extending automation, improving quality control, optimizing supply chain management, and furthering sustainability, AI has become a torchbearer to define new processes of production for the modern world.
Far from being hype, AI is essential for competitiveness, enhancing efficiency, productivity, and adaptability. Key benefits include improved quality control through accurate defect detection, predictive maintenance to reduce downtime, optimized resource use for sustainability, better inventory and demand forecasting, and safer workplaces with AI-driven robotics handling hazardous tasks. Together, these advantages make AI a powerful tool for increasing productivity, quality, and safety in manufacturing.
Need help with a manufacturing project?
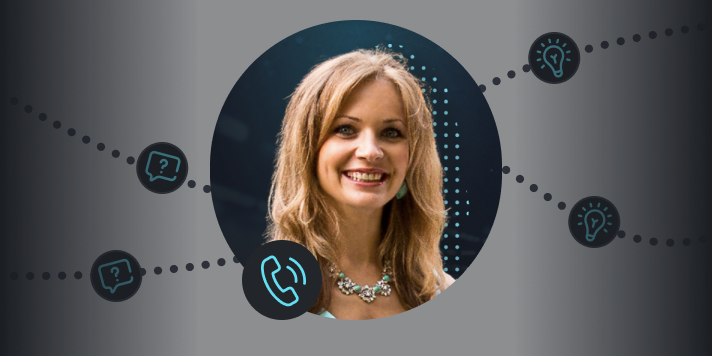
Talk to Yuliya. She will make sure that all is covered. Don't waste time on googling - get all answers from relevant expert in under one hour.